The convergence of artificial intelligence and human expertise is creating unprecedented opportunities for profit and innovation across virtually every industry. As AI technologies continue to evolve at a breathtaking pace, the most successful businesses aren't simply replacing humans with automation—they're finding the sweet spots where AI and human capabilities complement and enhance each other.
In today's rapidly evolving technological landscape, understanding where these profitable intersections exist is crucial for businesses seeking to maximize returns on their AI investments. The global AI market is projected to reach $826 billion by 2030, according to recent forecasts [1], but the distribution of these gains will be far from equal. Organizations that strategically identify and capitalize on the most lucrative AI-human intersections will capture disproportionate value.
This article explores nine key areas where the combination of AI capabilities and human expertise is generating exceptional profit potential. From content creation and education to healthcare and finance, we'll examine market sizes, growth projections, real-world success stories, and actionable insights to help you navigate these opportunities.
Whether you're an entrepreneur looking for your next venture, an executive planning strategic investments, or a professional seeking to future-proof your career, understanding these high-value intersections will provide you with a roadmap to capitalize on the AI revolution. The future belongs not to AI alone, nor to human expertise in isolation, but to those who master the art of bringing them together in ways that create maximum value.
Let's explore the nine most profitable intersections of AI and human expertise, backed by market data, case studies, and expert insights that will help you position your business or career for success in the AI-augmented economy.
Content and Information: The Foundation of AI Value
In today's AI-driven economy, content and information have emerged as the bedrock upon which artificial intelligence builds its value. AI models constantly seek fresh, high-quality content to learn from, making this intersection one of the most consistently profitable areas for businesses and entrepreneurs.
The Growing Market for AI-Ready Content
The global market for AI content creation and management is projected to reach $47.5 billion by 2026, with a compound annual growth rate (CAGR) of 29.8% according to recent industry analyses [2]. This explosive growth reflects the insatiable demand for quality content that can feed AI systems and enhance their capabilities.
What makes this intersection particularly valuable is its fundamental nature—AI systems require vast amounts of well-structured, accurate information to function effectively. As one industry expert puts it, "Data is the new oil, but curated, high-quality content is the refined fuel that powers advanced AI systems."
Why AI Needs Human-Created Content
Despite significant advances in generative capabilities, AI still relies heavily on human-created content for several critical reasons:
Training data quality directly impacts AI performance. Models trained on comprehensive, diverse, and accurate datasets consistently outperform those trained on lower-quality information. This creates a premium market for specialized content creation services that can provide high-quality training data.
Domain expertise remains irreplaceable. In specialized fields like medicine, law, and engineering, human experts provide the nuanced understanding that makes AI applications valuable. Companies that can effectively capture and structure this expertise create significant competitive advantages.
Ethical considerations and bias mitigation require human oversight. As organizations increasingly prioritize responsible AI development, human-in-the-loop content creation and curation services have seen growing demand, with some specialized providers reporting revenue growth exceeding 200% year-over-year [3].
Profitable Business Models at This Intersection
Several business models have proven particularly successful at the AI-content intersection:
Specialized Content Creation Services: Companies like Narrative Science have built profitable businesses by creating customized, AI-ready content for specific industries. Their approach combines domain expertise with an understanding of AI requirements, commanding premium pricing for specialized content creation.
Content Optimization Platforms: Tools that help optimize existing content for AI consumption are seeing rapid adoption. ClickUp, for example, used SurferSEO to grow their blog traffic by 85% over 12 months by optimizing content for both human readers and AI systems [4].
Data Annotation and Labeling Services: Scale AI reached a valuation of $7.3 billion by providing human-powered data annotation services that prepare content for AI training. Their success demonstrates the enormous value of the human touch in making content AI-ready.
Content Repurposing Solutions: AI-powered tools that help repurpose existing content across formats and channels are showing strong growth. According to Netguru's 2025 analysis, companies like Repurpose.io have achieved 150% revenue growth by helping businesses maximize the value of their content assets through AI-driven repurposing [5].
Real-World Success Stories
The theoretical potential of this intersection is being realized through numerous success stories:
The New York Times has developed a sophisticated content strategy that leverages both human journalism and AI tools. Their approach includes creating structured data from their vast archives, making it accessible for AI analysis while preserving the human expertise that gives their content value. This strategy has contributed to a 30% increase in digital subscription revenue.
Mayo Clinic has created a profitable venture by structuring and licensing their medical knowledge base for AI applications. By combining their world-class medical expertise with AI-ready content formatting, they've created a new revenue stream while advancing healthcare AI.
Bloomberg has successfully monetized financial data through their AI-ready content services. Their approach combines human financial expertise with structured data that's optimized for AI consumption, creating high-value products for the financial sector.
Future Trends and Opportunities
Looking ahead, several trends are likely to further increase the value of this intersection:
Multimodal content that combines text, images, audio, and video in AI-ready formats will command premium prices as AI systems become more sophisticated in processing diverse content types.
Synthetic data generation guided by human experts will grow as organizations seek to supplement real-world data with artificially created scenarios, particularly in sensitive or rare-case domains.
Specialized content marketplaces that connect domain experts with AI developers will expand, creating new opportunities for knowledge monetization.
Content authentication services will become increasingly valuable as the need to verify the provenance and accuracy of AI training data grows in importance.
Strategic Considerations for Businesses
For organizations looking to capitalize on this intersection, several strategic considerations should guide their approach:
- Identify domains where your organization has unique content or expertise that could be valuable for AI applications.
- Invest in structuring your content in AI-ready formats that preserve context and relationships.
- Consider partnerships with AI developers who need specialized content in your domain.
- Develop clear policies around content ownership, usage rights, and compensation models.
- Build capabilities that combine domain expertise with an understanding of AI requirements.
The intersection of content, information, and AI represents one of the most durable profit opportunities in the AI landscape. As AI continues to advance, the value of high-quality, well-structured content will only increase, creating sustained opportunities for businesses that can effectively bridge the human-AI content gap.
Education and Training: The AI Knowledge Economy
The demand for understanding and effectively applying AI in business and career contexts has created one of the most lucrative intersections in the AI landscape. As organizations race to implement AI solutions, the need for skilled professionals and comprehensive training programs has skyrocketed.
Market Size and Explosive Growth
The AI education and training market is experiencing unprecedented expansion. According to Grand View Research, the global AI in education market size was valued at $3.3 billion in 2024 and is projected to reach $20.8 billion by 2032, growing at a CAGR of 36.0% from 2025 to 2032 [6]. This remarkable growth reflects the urgent need for AI literacy across virtually every industry and profession.
The demand spans multiple segments, from technical AI skills development to executive education on AI strategy. As one education industry analyst notes, "We're seeing a fundamental shift where AI literacy is becoming as essential as digital literacy was a decade ago—but the learning curve is steeper and the stakes are higher."
Why Education Represents a Prime Profit Opportunity
Several factors make education and training a particularly profitable AI-human intersection:
Persistent skills gap: Despite growing demand, qualified AI professionals remain scarce. According to industry reports, over 70% of organizations struggle to find employees with adequate AI skills, creating premium pricing opportunities for effective training solutions.
Continuous learning requirements: The rapid evolution of AI technologies necessitates ongoing education, creating recurring revenue streams rather than one-time transactions. As models, tools, and applications evolve, professionals must regularly update their knowledge.
Cross-industry relevance: Unlike some specialized technologies, AI applications span virtually every sector, creating a massive addressable market for education providers who can tailor their offerings to different industries.
High stakes implementation: The significant business impact of AI implementations—both positive and negative—creates strong incentives for organizations to invest in quality training. Failed AI initiatives due to lack of expertise can cost companies millions, making education a high-ROI investment.
Profitable Business Models in AI Education
Several business models have proven particularly successful in the AI education space:
Specialized AI bootcamps and certification programs: Intensive, focused training programs that develop practical AI skills command premium prices. For example, specialized AI engineering bootcamps can charge $10,000-$20,000 for 12-16 week programs, with some reporting placement rates above 85% and average starting salaries exceeding $110,000.
Corporate AI training programs: Customized training solutions for enterprises represent a high-margin opportunity. Companies like General Assembly and Udacity have built nine-figure businesses largely through corporate training contracts for emerging technologies including AI.
AI curriculum licensing: Developing and licensing AI curriculum to educational institutions has emerged as a scalable model. Companies providing turnkey AI education solutions to universities report 40-60% gross margins and rapid growth as institutions rush to offer AI programs.
AI education platforms: Subscription-based platforms offering continuously updated AI training content create valuable recurring revenue streams. Leading platforms report subscriber retention rates above 70% and growing average revenue per user as professionals seek to maintain current AI knowledge.
Executive education on AI strategy: Programs helping leaders understand AI's strategic implications command premium pricing. Top business schools report their AI-focused executive education programs are among their highest-revenue offerings, with some charging $25,000+ for week-long immersive programs.
Real-World Success Stories
The theoretical potential of this intersection is being realized through numerous success stories:
Coursera's AI specializations, developed in partnership with deeplearning.ai and leading universities, have attracted millions of learners. Their machine learning course taught by Andrew Ng became one of the most popular courses in the platform's history, demonstrating the massive demand for quality AI education [7].
Georgia Tech's Online Master of Science in Computer Science with AI specialization has enrolled over 11,000 students, generating significant revenue while addressing the AI skills gap. Their innovative approach combines academic rigor with accessibility, creating a scalable model for AI higher education.
DataCamp has built a successful business focused on data science and AI skills development, with over 9 million learners. Their subscription model provides continuous learning opportunities as AI technologies evolve, creating strong recurring revenue.
O'Reilly Media has successfully pivoted to focus heavily on AI education content and events, with their AI conferences becoming major revenue generators. Their approach combines multiple formats—books, videos, interactive learning, and live events—to create comprehensive AI learning experiences.
DeepLearning.AI has created a profitable education company focused exclusively on AI training, with courses reaching hundreds of thousands of learners. Their success demonstrates the viability of specialized AI education providers in a crowded education market.
Future Trends and Opportunities
Looking ahead, several trends are likely to further increase the value of this intersection:
AI-powered personalized learning will create a virtuous cycle where AI improves the effectiveness of AI education itself. Adaptive learning platforms that customize content based on individual progress show 20-30% better outcomes than traditional approaches.
Simulation and hands-on learning environments will become increasingly important as organizations seek practical AI skills. Virtual labs and sandboxed environments that allow risk-free experimentation with AI systems represent a growing opportunity.
Industry-specific AI training will command premium prices as organizations seek contextually relevant education. Programs tailored to healthcare, finance, manufacturing, and other sectors can charge 30-50% more than generic AI training.
AI ethics and governance education will see growing demand as regulatory scrutiny increases. Organizations are increasingly willing to invest in training that helps them implement AI responsibly and avoid costly missteps.
Continuous AI education subscriptions will become standard as professionals recognize the need for ongoing learning. Models that combine foundational training with regular updates on emerging techniques show strong retention and lifetime value.
Strategic Considerations for Businesses
For organizations looking to capitalize on this intersection, several strategic considerations should guide their approach:
- Focus on practical, applicable knowledge rather than purely theoretical concepts. The highest-value education bridges theory and practice.
- Develop industry-specific content that addresses the unique AI challenges and opportunities in particular sectors.
- Build credibility through partnerships with recognized AI experts, academic institutions, or industry leaders.
- Create clear pathways from learning to application, helping students translate knowledge into career advancement.
- Invest in continuous content updates to keep pace with the rapidly evolving AI landscape.
The intersection of AI and education represents one of the most sustainable profit opportunities in the current technology landscape. As AI continues to transform industries, the demand for effective education will only grow, creating enduring opportunities for businesses that can effectively bridge the knowledge gap.
Business Integration: AI as a Competitive Advantage
Companies across all industries are actively seeking ways to integrate AI into their processes to increase efficiency, reduce costs, and gain competitive advantages. This intersection represents one of the most immediate and substantial profit opportunities in the AI landscape.
Market Size and Rapid Expansion
The global market for AI business integration is experiencing explosive growth. According to Precedence Research, the global artificial intelligence market size was valued at $196.6 billion in 2023 and is projected to surpass $1.8 trillion by 2030, growing at a CAGR of 37.3% from 2023 to 2030 [8]. This remarkable expansion reflects the urgent priority businesses place on AI adoption and integration.
What makes this intersection particularly valuable is its direct impact on business performance metrics. As one industry analyst notes, "AI integration isn't just about technology adoption—it's fundamentally about transforming business economics through automation, prediction, and optimization."
Why Business Integration Creates Exceptional Value
Several factors make business integration a particularly profitable AI-human intersection:
Immediate ROI potential: Unlike some AI applications that require years to show returns, well-executed business integrations can deliver measurable results within months. Companies report cost reductions of 15-40% in targeted processes through AI automation.
Competitive necessity: As AI adoption accelerates, organizations that fail to integrate these technologies risk falling behind competitors who achieve greater efficiency and innovation. This competitive pressure creates urgency and willingness to invest.
Scalable impact: AI solutions that successfully address one business challenge can often be expanded to related processes, creating multiplier effects on the initial investment. This scalability significantly enhances the lifetime value of AI integration projects.
Cross-functional benefits: AI integration frequently delivers unexpected benefits beyond the initial use case, as insights and capabilities developed for one purpose prove valuable elsewhere in the organization.
Profitable Business Models in AI Integration
Several business models have proven particularly successful in the AI business integration space:
AI implementation consulting: Specialized consultancies that help organizations identify, implement, and optimize AI solutions command premium rates. Leading firms report average project values of $500,000 to $2 million for comprehensive AI integration initiatives.
Industry-specific AI solutions: Vertical-focused AI products that address the unique challenges of specific industries achieve higher adoption rates and command premium pricing. Solutions tailored to manufacturing, healthcare, financial services, and retail have shown particularly strong traction.
AI integration platforms: Tools that simplify the process of connecting AI capabilities with existing business systems create valuable efficiency gains. These platforms can reduce integration time by 40-60% compared to custom development approaches.
Process-specific AI solutions: AI tools focused on specific business processes like customer service, procurement, or inventory management deliver targeted value. These solutions can achieve adoption rates 3-5x higher than general-purpose AI platforms.
AI transformation programs: Comprehensive programs that combine technology, process redesign, and organizational change management create sustainable competitive advantages. These programs typically span 12-36 months and represent significant investments with correspondingly high returns.
Real-World Success Stories
The theoretical potential of this intersection is being realized through numerous success stories:
Aberdeen City Council implemented Microsoft 365 Copilot as a holistic, AI-driven solution to offload tasks and free up workforce capacity for resident care. The results were impressive, with a projected 241% ROI in time savings and improved productivity, saving an estimated $3 million annually [9].
Allpay accelerated code development and information sharing by implementing GitHub Copilot for engineers and developers, and Microsoft Copilot for SharePoint. This strategic integration increased productivity by 10% and delivery volume into production by 25%.
ANS transformed their selling process by implementing Microsoft Copilot and agents to streamline operations. The AI solution collects and summarizes information across data sources, providing insights from previous customer interactions. This integration is forecast to increase closing ratio by 6.25% by allowing sellers to prioritize time on critical accounts.
Architecht dramatically improved UI/UX prototyping and development using Azure OpenAI Service and GitHub Copilot to develop their OBA Suite cloud-based platform. The results were transformative: UI/UX prototyping now takes just 25 minutes instead of two days, with enhanced user experience through AI-driven assistants.
British Columbia Investment Management Corporation (BCI) addressed manual tasks that were reducing productivity and employee satisfaction by implementing Microsoft 365 Copilot and Azure ecosystem. The results showed increased productivity by 10-20% for 84% of Copilot users, increased job satisfaction by 68%, and saved over 2,300 person-hours through automation.
Future Trends and Opportunities
Looking ahead, several trends are likely to further increase the value of this intersection:
AI orchestration will become increasingly important as organizations deploy multiple AI solutions that need to work together seamlessly. Platforms that can coordinate diverse AI capabilities will command premium prices.
Embedded AI that integrates artificial intelligence directly into core business applications will accelerate adoption by reducing implementation friction. This approach can increase AI utilization by 3-5x compared to standalone solutions.
Democratized AI tools that enable non-technical business users to create and deploy AI solutions will expand the addressable market. These tools can increase the number of AI use cases in an organization by an order of magnitude.
AI governance frameworks will become essential as organizations scale their AI implementations. Solutions that help manage AI risk, ensure compliance, and maintain quality will see growing demand.
Industry-specific AI ecosystems that combine data, models, and applications tailored to particular sectors will create powerful network effects and sustainable competitive advantages.
Strategic Considerations for Businesses
For organizations looking to capitalize on this intersection, several strategic considerations should guide their approach:
- Focus on business outcomes rather than technology capabilities, aligning AI integration with specific performance metrics.
- Develop deep understanding of industry-specific processes and challenges to create contextually relevant solutions.
- Build capabilities that bridge technical AI expertise with business process knowledge.
- Create clear methodologies for identifying, prioritizing, and implementing AI use cases.
- Invest in change management capabilities to ensure successful adoption of AI-driven processes.
The intersection of AI and business integration represents one of the most immediate and substantial profit opportunities in the current technology landscape. As organizations increasingly recognize AI as a competitive necessity rather than a technological novelty, the demand for effective integration solutions will continue to grow, creating enduring opportunities for businesses that can effectively bridge the gap between AI capabilities and business value.
AI in Legal Field: Transforming Justice and Compliance
The intersection of AI and legal services represents one of the most profitable and rapidly evolving opportunities in the professional services sector. From automating contract analysis to revolutionizing legal research, AI is fundamentally changing how legal work is performed and valued.
Market Size and Accelerating Growth
The global legal AI market is experiencing remarkable expansion. According to Grand View Research, the legal AI market size was valued at $1.3 billion in 2024 and is projected to reach $9.5 billion by 2032, growing at a CAGR of 28.6% from 2025 to 2032 [10]. This exceptional growth reflects the legal industry's increasing recognition of AI's potential to transform traditionally labor-intensive processes.
What makes this intersection particularly valuable is its ability to address the persistent challenges of legal service delivery: high costs, time constraints, and access limitations. As one legal technology expert notes, "AI in law isn't just about efficiency—it's about fundamentally reimagining how legal services can be delivered, priced, and accessed."
Why Legal AI Creates Exceptional Value
Several factors make the legal field a particularly profitable AI-human intersection:
High-value knowledge work: Legal services traditionally command premium rates due to the specialized expertise required. AI that can automate aspects of this work while maintaining quality creates significant value.
Document-intensive processes: The legal profession involves processing vast amounts of text-based information—contracts, case law, regulations, and more. This text-heavy environment is ideally suited to AI's natural language processing capabilities.
Risk mitigation imperative: Organizations face growing regulatory complexity and legal risks, creating strong incentives to invest in AI solutions that improve compliance and reduce exposure.
Access to justice gap: Traditional legal service models leave many individuals and small businesses unable to afford necessary legal help. AI solutions that reduce costs can tap into this underserved market.
Profitable Business Models in Legal AI
Several business models have proven particularly successful in the legal AI space:
Contract analysis platforms: AI-powered tools that automate contract review, extraction, and management are seeing rapid adoption. Leading platforms report 60-80% time savings compared to manual review, with some enterprise clients saving millions annually in legal costs.
Legal research augmentation: AI systems that enhance legal research capabilities by analyzing case law, statutes, and regulations command premium subscription fees. These platforms can reduce research time by 70% while improving thoroughness.
Regulatory compliance solutions: AI tools that monitor regulatory changes and assess compliance risks address a critical pain point for organizations. These solutions can reduce compliance costs by 30-50% while improving risk management.
Litigation analytics: Platforms that analyze historical case outcomes, judicial tendencies, and opposing counsel strategies provide valuable strategic insights. Some litigation analytics tools report helping clients improve settlement outcomes by 15-25%.
Document automation: AI-powered systems that generate legal documents based on specific parameters and requirements dramatically reduce drafting time. These solutions can cut document preparation costs by 50-90% for routine matters.
Real-World Success Stories
The theoretical potential of this intersection is being realized through numerous success stories:
AI Agents for Contract Analysis are becoming the next big trend driving automation of legal workflows. These advanced systems can automatically analyze contracts and compare with predefined criteria, identify and evaluate relevant clauses, independently provide feedback and suggestions for changes, interact with CRM or ERP systems, and support contract negotiations by optimizing legal arguments [11]. Unlike traditional static analysis tools, these AI agents take a proactive and dynamic approach to contract management.
Explainable AI in Legal Applications is addressing the "black box" problem of traditional AI systems. By making AI decision-making processes transparent, lawyers can understand what data and patterns AI models use, promoting trust in the technology and helping adhere to legal regulations and compliance standards. This transparency ensures automated processes comply with current legal requirements.
Multilingual Contract Analysis is solving a critical challenge for global companies dealing with contracts in multiple languages. AI-powered multilingual analysis reduces the need for human translators and ensures consistent contract interpretation across languages, creating significant efficiency gains for international organizations.
Law Firms Using AI for Risk Analysis are leveraging machine learning algorithms to predict potential legal problems based on historical data. This approach enables improved risk management and proactive legal strategy, helping clients avoid costly disputes before they arise.
AI-Powered Legal Research and Case Analysis tools are transforming how lawyers prepare for cases. By improving research and analysis processes, these tools enable faster, more comprehensive legal research capabilities, allowing lawyers to focus on strategy and client service rather than tedious document review.
Future Trends and Opportunities
Looking ahead, several trends are likely to further increase the value of this intersection:
AI judges and mediators for certain types of disputes will emerge as viable alternatives to traditional adjudication. Early experiments with AI-assisted mediation show resolution rates comparable to human mediators for certain dispute types, with significantly lower costs.
Predictive justice capabilities will become more sophisticated, enabling more accurate outcome predictions based on case facts, jurisdiction, and assigned judge. These tools will transform litigation strategy and settlement negotiations.
Regulatory AI that can not only monitor compliance but proactively suggest policy and process changes to address regulatory risks will command premium prices. These systems will evolve from monitoring tools to strategic advisors.
Smart contracts with embedded legal intelligence will create new business models at the intersection of law, finance, and technology. These contracts will increasingly incorporate AI-driven risk assessment and automated dispute resolution mechanisms.
Legal data marketplaces will emerge as valuable platforms connecting those with legal data to those building AI legal applications. These marketplaces will accelerate innovation while creating new monetization opportunities for legal information.
Strategic Considerations for Businesses
For organizations looking to capitalize on this intersection, several strategic considerations should guide their approach:
- Focus on augmenting rather than replacing legal professionals, creating tools that enhance human capabilities rather than attempting to fully automate complex legal judgment.
- Develop deep domain expertise in specific legal practice areas or industries where AI can add particular value.
- Address data privacy and security concerns proactively, as legal information is often highly sensitive.
- Build explainable AI that can provide reasoning for its conclusions, which is particularly important in legal contexts.
- Invest in user experience design that makes sophisticated AI capabilities accessible to legal professionals with varying levels of technical expertise.
The intersection of AI and legal services represents one of the most promising profit opportunities in the professional services landscape. As regulatory complexity continues to increase and organizations seek more efficient legal solutions, the demand for effective legal AI will continue to grow, creating enduring opportunities for businesses that can effectively bridge the gap between legal expertise and AI capabilities.
AI in Healthcare: Precision and Efficiency
The integration of AI into healthcare represents one of the most promising and potentially lucrative intersections in the technological landscape. With substantial funding and high demand for solutions that improve diagnostic accuracy and operational efficiency, healthcare AI is poised for exceptional growth and profitability.
Market Size and Explosive Growth
The global AI in healthcare market is experiencing remarkable expansion. According to Grand View Research, the market size was valued at $15.4 billion in 2024 and is projected to reach $187.9 billion by 2032, growing at a staggering CAGR of 37.5% from 2025 to 2032 [12]. This exceptional growth reflects the healthcare industry's recognition of AI's potential to transform patient care while addressing persistent challenges of cost, access, and quality.
What makes this intersection particularly valuable is its direct impact on human health and wellbeing, combined with the enormous scale of healthcare spending globally. As one healthcare technology expert notes, "AI in healthcare isn't just about efficiency—it's about fundamentally improving outcomes while making quality care more accessible and affordable."
Why Healthcare AI Creates Exceptional Value
Several factors make healthcare a particularly profitable AI-human intersection:
High-stakes decisions: Healthcare involves critical decisions where improved accuracy has enormous value. AI that can enhance diagnostic precision or treatment selection creates significant benefits for patients and providers alike.
Data-rich environment: Healthcare generates vast amounts of complex data—from medical images and electronic health records to genomic information and wearable device outputs. This data-rich context is ideal for AI applications.
Persistent efficiency challenges: Healthcare systems worldwide struggle with rising costs and resource constraints. AI solutions that improve operational efficiency address urgent needs for healthcare organizations.
Personalization imperative: Modern healthcare increasingly recognizes the importance of personalized approaches. AI enables cost-effective personalization at scale that would be impossible through human efforts alone.
For example:
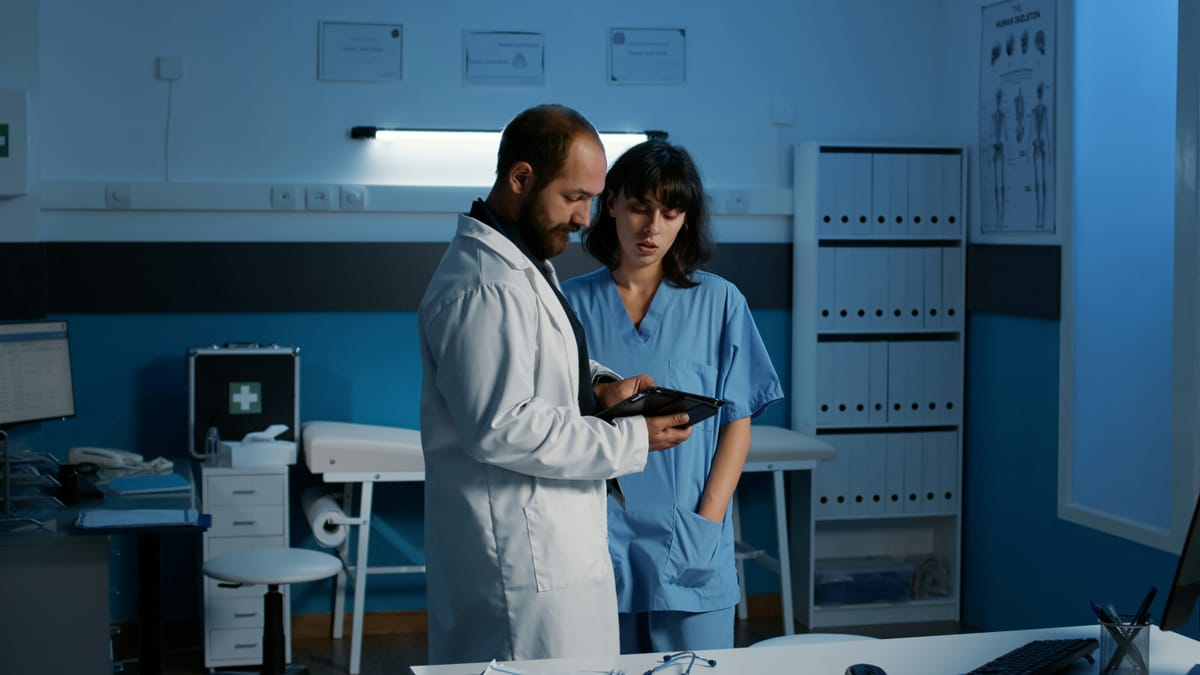
Profitable Business Models in Healthcare AI
Several business models have proven particularly successful in the healthcare AI space:
Diagnostic support systems: AI-powered tools that assist clinicians in detecting diseases from medical images or patient data command premium prices. Leading platforms report 30-50% improvements in early detection rates for certain conditions, creating enormous value for healthcare systems.
Clinical decision support: AI systems that analyze patient data to recommend treatment options or flag potential issues are seeing rapid adoption. These solutions can reduce treatment variability and improve adherence to best practices by 40-60%.
Operational optimization: AI tools that improve hospital operations, resource allocation, and patient flow address critical efficiency challenges. Some solutions report reducing patient wait times by 25-35% while improving resource utilization.
Remote monitoring platforms: AI-enhanced systems that monitor patients outside clinical settings enable earlier interventions and reduced hospitalizations. These platforms can decrease hospital readmissions by 15-30% for certain chronic conditions.
Drug discovery acceleration: AI platforms that enhance pharmaceutical research and development processes are commanding billion-dollar valuations. These solutions can reduce drug discovery timelines by years and significantly increase success rates.
Real-World Success Stories
The theoretical potential of this intersection is being realized through numerous success stories:
Massachusetts General Hospital (MGH) and MIT collaborated to develop AI algorithms for radiology applications. Their AI system achieved 94% diagnostic accuracy in detecting lung nodules, significantly outperforming human radiologists who scored 65% accuracy in the same task [13]. This implementation relieved radiologists of mundane tasks, streamlined the diagnostic process, expedited treatment initiation, and enhanced patient outcomes.
Mayo Clinic and IBM Watson Health created AI-driven tools for personalized medicine. Watson's cognitive computing analyzes patient data including genetic profiles, medical records, treatment histories, and scientific studies to provide tailored treatment recommendations. The results showed higher response rates, longer progression-free survival, and improved overall outcomes compared to standard treatments, while also increasing patient satisfaction and reducing healthcare expenses.
Cerner Corporation and University of Missouri Health Care integrated AI algorithms into Electronic Health Records systems to automate routine tasks and enhance data analytics capabilities. This implementation reduced administrative burden, improved clinical decision-making, enhanced healthcare outcomes, improved patient safety by flagging potential errors, and facilitated data-driven healthcare delivery.
AI-Assisted Surgical Robotics combines AI technologies with surgical robotics to guide procedures with enhanced precision, reduced invasiveness, and accelerated patient recovery. This technology has improved surgical outcomes, reduced complications, and shortened hospital stays.
AI Diagnostics for Early Disease Detection uses algorithms to analyze tissue samples, blood tests, and laboratory results with remarkable precision, helping pathologists identify abnormalities that might otherwise be missed. This capability enables earlier disease detection and improved treatment outcomes.
Future Trends and Opportunities
Looking ahead, several trends are likely to further increase the value of this intersection:
Multimodal AI diagnostics that integrate information from diverse sources—imaging, lab tests, patient history, genomics—will enable more comprehensive and accurate assessments than any single data type could provide.
Ambient clinical intelligence that can automatically document patient encounters will address the growing documentation burden that consumes up to 50% of clinician time. These solutions can increase provider productivity by 15-25%.
Precision medicine platforms that match patients with optimal treatments based on their unique characteristics will transform care for complex conditions. Early implementations show 30-40% improvements in treatment response rates for certain diseases.
Predictive healthcare analytics that identify patients at risk for deterioration or readmission will enable proactive interventions. These systems can reduce adverse events by 20-30% through earlier detection and response.
AI-enabled clinical trials that optimize patient selection, monitoring, and data analysis will accelerate medical innovation. These approaches can reduce trial timelines by 30-50% while improving success rates.
Strategic Considerations for Businesses
For organizations looking to capitalize on this intersection, several strategic considerations should guide their approach:
- Focus on clinical validation and evidence generation, as healthcare adoption requires demonstrated efficacy and safety.
- Address integration challenges proactively, designing solutions that work within existing clinical workflows and IT infrastructures.
- Develop clear regulatory strategies, as healthcare AI faces increasing oversight from agencies like the FDA and EMA.
- Build multidisciplinary teams that combine clinical, technical, and regulatory expertise.
- Invest in explainable AI that can provide reasoning for its conclusions, which is particularly important in clinical contexts.
The intersection of AI and healthcare represents one of the most promising profit opportunities in the technology landscape. As healthcare systems worldwide seek solutions to improve outcomes while controlling costs, the demand for effective healthcare AI will continue to grow, creating enduring opportunities for businesses that can effectively bridge the gap between clinical needs and AI capabilities.
Creative AI Content: Art, Music, and Innovation
The fusion of AI with creative industries is dramatically reshaping how content is produced, distributed, and monetized. This intersection represents one of the most dynamic and potentially lucrative opportunities in the AI landscape, with significant implications for film, music, visual arts, and other creative fields.
If you would like to find out more detailed information about artificial intelligence generation platforms, you can access and read additional insights on this subject by referring to our topic titled Generative AI Platforms, which covers this area in greater depth.
Market Size and Rapid Expansion
The global market for AI in creative content is experiencing explosive growth. According to Grand View Research, the generative AI in music market alone was valued at $229 million in 2023 and is projected to reach $2.6 billion by 2032, growing at a CAGR of 31.2% from 2024 to 2032 [14]. The broader AI creative content market, encompassing visual arts, film, and other media, is expanding at a similar pace.
What makes this intersection particularly valuable is its ability to dramatically reduce production costs while enabling new forms of creative expression. As one industry analyst notes, "AI in creative fields isn't just about automation—it's about unlocking entirely new possibilities for human creativity while making production more accessible and affordable."
Why Creative AI Creates Exceptional Value
Several factors make creative content a particularly profitable AI-human intersection:
Production cost reduction: Creative content production traditionally involves significant time and resource investments. AI tools that automate aspects of this work while maintaining quality create immediate value through cost savings of 50-80% for certain tasks.
Democratization of creation: AI is lowering barriers to entry for creative production, enabling individuals and small teams to produce professional-quality content without large budgets or specialized technical skills. This expands the market for creative tools dramatically.
Personalization at scale: AI enables the cost-effective creation of personalized or customized creative content that would be prohibitively expensive through traditional methods. This capability opens new business models and revenue streams.
Creative augmentation: Rather than replacing human creativity, AI tools are increasingly focused on augmenting human capabilities, helping creators overcome technical limitations or creative blocks. This collaborative approach creates value for professional creators.
Profitable Business Models in Creative AI
Several business models have proven particularly successful in the creative AI space:
AI-powered creative tools: Software that uses AI to simplify or enhance creative processes commands premium subscription fees. Leading platforms report 200-300% year-over-year growth as creators embrace these productivity-enhancing tools.
Content generation platforms: Services that generate customized creative assets—from music and images to video and text—are seeing rapid adoption. These platforms can reduce content creation costs by 60-90% compared to traditional production methods.
Licensing and rights management: AI systems that manage the increasingly complex landscape of creative rights and licensing create significant value. These solutions help creators monetize their work more effectively while reducing legal risks.
Creative marketplaces: Platforms that connect AI-assisted creators with clients or audiences are creating new economic opportunities. Some marketplaces report that AI-enhanced creators earn 30-50% more than those using traditional methods.
Personalized entertainment: AI-driven systems that customize entertainment experiences based on individual preferences are showing strong growth. These solutions can increase engagement metrics by 40-60% compared to non-personalized content.
Real-World Success Stories
The theoretical potential of this intersection is being realized through numerous success stories:
The "Portrait of Edmond de Belamy" became the first artwork created by AI to be sold at a major auction house (Christie's) for $432,500 in 2018 [15]. This sale marked a pivotal moment in the art world's recognition of AI as a creative force and opened new markets for AI-generated art.
Refik Anadol's immersive installations use GANs and vast datasets to create mesmerizing visual and audio experiences. His projects include "Machine Hallucination," which processes millions of images of New York City to generate dynamic, data-driven visualizations, and "Artificial Realities: Coral," commissioned by the World Economic Forum to raise awareness about climate change. These works demonstrate how AI can transform data into emotionally resonant artistic experiences.
Sofia Crespo's "Neural Zoo" explores speculative life forms using natural and AI data to create stunning visuals of imagined nature. Her work reflects the mechanisms of human creativity while pushing the boundaries of what's possible through human-AI collaboration.
BMW's digital art campaign showcased the commercial application of AI in creative contexts. By training AI on 900 years of art history to generate 50 new artworks that were projection-mapped onto a virtual car, BMW created a unique marketing campaign that garnered significant attention and engagement.
Nutella's unique jar labels campaign used AI to create seven million unique jar labels, generating curiosity and increased demand for their product. This mass customization would have been impossible without AI technology and demonstrates how creative AI can drive tangible business results.
Google's DeepDream, an early exploration of AI in art, utilizes algorithmic pareidolia to create dream-like, psychedelic versions of existing images. This technology inspired further research into AI's creative potential and has influenced a generation of AI artists.
Future Trends and Opportunities
Looking ahead, several trends are likely to further increase the value of this intersection:
Collaborative AI that works alongside human creators as an active partner rather than a passive tool will transform creative workflows. These systems will learn individual creators' styles and preferences, becoming increasingly valuable creative partners over time.
Multimodal generation that can simultaneously work across different media types—creating coordinated music, visuals, and text—will enable more integrated creative production. Early implementations show 40-60% efficiency gains in multimedia projects.
Emotional intelligence in creative AI will enable more nuanced and impactful content generation. Systems that can understand and evoke specific emotional responses will command premium prices in advertising and entertainment.
Decentralized creative economies built on blockchain technology will create new ways for AI-assisted creators to monetize their work. These models could increase creator earnings by 20-40% by reducing intermediaries.
Real-time adaptive content that evolves based on audience response will transform entertainment and advertising. These systems can increase engagement by 30-50% compared to static content.
Strategic Considerations for Businesses
For organizations looking to capitalize on this intersection, several strategic considerations should guide their approach:
- Focus on human-AI collaboration rather than replacement, creating tools that enhance human creativity rather than attempting to fully automate creative processes.
- Address ethical and copyright concerns proactively, as creative AI faces increasing scrutiny regarding training data sources and attribution.
- Develop domain expertise in specific creative fields to ensure AI tools address genuine creator needs and workflows.
- Build communities around creative AI tools to accelerate adoption and gather valuable feedback for improvement.
- Invest in user experience design that makes sophisticated AI capabilities accessible to creators with varying levels of technical expertise.
The intersection of AI and creative content represents one of the most dynamic and potentially transformative profit opportunities in the current technology landscape. As AI continues to evolve from a technical curiosity to an essential creative tool, the demand for effective creative AI solutions will continue to grow, creating enduring opportunities for businesses that can effectively bridge the gap between technical capabilities and creative expression.
AI for Finance and Investments: Smart Money
The integration of AI into financial services and investment management represents one of the most mature and profitable intersections in the AI landscape. With massive budgets and high-stakes decisions, the finance sector has been an early and enthusiastic adopter of AI technologies.
Market Size and Substantial Growth
The global AI in finance market is experiencing robust expansion. According to Markets and Markets, the market size was valued at $16.5 billion in 2024 and is projected to reach $42.6 billion by 2028, growing at a CAGR of 26.8% [16]. This significant growth reflects the financial industry's recognition of AI's potential to transform everything from risk assessment to trading strategies.
What makes this intersection particularly valuable is the direct impact on financial outcomes and the enormous scale of the global financial system. As one fintech analyst notes, "AI in finance isn't just about efficiency—it's about making better decisions with trillions of dollars at stake, where even marginal improvements create enormous value."
Why Financial AI Creates Exceptional Value
Several factors make finance a particularly profitable AI-human intersection:
Data-driven decision making: Financial services have always relied heavily on data analysis, making them natural candidates for AI enhancement. AI systems can process vastly more information than human analysts, identifying patterns and opportunities that would otherwise be missed.
High-value transactions: The sheer scale of financial transactions means that even small improvements in efficiency or accuracy create substantial value. AI systems that improve trading outcomes by even fractions of a percent can generate millions in additional returns.
Risk management imperative: Financial institutions face complex risk landscapes that AI can help navigate more effectively. Solutions that better predict and mitigate risks command premium prices due to their direct impact on the bottom line.
Regulatory complexity: Financial services operate in highly regulated environments that create significant compliance burdens. AI that can streamline compliance while reducing risk creates immediate ROI.
Profitable Business Models in Financial AI
Several business models have proven particularly successful in the financial AI space:
Algorithmic trading platforms: AI-powered systems that execute trades based on market data and predictive models are seeing widespread adoption. Leading platforms report 15-30% performance improvements compared to traditional approaches, creating enormous value for investment firms.
Risk assessment solutions: AI tools that evaluate credit risk, fraud potential, or investment risk more accurately than traditional methods command premium prices. These solutions can reduce default rates by 20-40% while expanding access to financial services.
Personalized financial advice: AI-driven platforms that provide customized financial guidance at scale are disrupting traditional wealth management. These solutions can reduce the cost of financial advice by 70-90% while improving accessibility.
Regulatory compliance automation: AI systems that monitor transactions, communications, and activities for compliance issues address a critical pain point for financial institutions. These platforms can reduce compliance costs by 30-50% while improving coverage.
Market intelligence platforms: AI-enhanced systems that analyze market trends, news, and data to provide actionable insights are creating competitive advantages for their users. Some solutions report helping clients identify investment opportunities 2-3x faster than manual methods.
Real-World Success Stories
The theoretical potential of this intersection is being realized through numerous success stories:
JP Morgan Chase implemented an advanced AI system to streamline loan approvals, analyzing credit history, transaction data, and financial behaviors to enhance creditworthiness assessments [17]. The results were impressive: loan processing times reduced from days to minutes/hours, enhanced customer satisfaction and loyalty, reduced operational expenses, and the ability to handle more loan applications without increasing staff.
Bank of America's Erica, an AI-powered virtual assistant for mobile banking, addresses evolving customer expectations for personalized, on-demand digital services. Erica provides personalized customer interaction with tailored banking advice, 24/7 availability for instant assistance, data-driven insights based on user transactions and behaviors, and improved operational efficiency by handling regular inquiries.
HSBC integrated an AI-driven system for anti-money laundering (AML) efforts to address the challenges traditional systems face with high volume and complex financial transactions. The implementation significantly increased detection of suspicious transactions, reduced false positives, improved compliance with evolving regulatory requirements, and reduced operational costs through automated surveillance.
Citibank implemented AI-powered chatbots for customer service to address high demand leading to long wait times and inconsistent experiences. The results showed improved response times, consistent service quality, and reduced operational costs.
Canoe Intelligence developed AI-powered investment decision-making tools that improved investment decision-making speed by 40% and delivered an average 8% increase in returns for clients, demonstrating the direct financial impact of well-implemented AI solutions.
Future Trends and Opportunities
Looking ahead, several trends are likely to further increase the value of this intersection:
Quantum AI for finance will enable more complex modeling and optimization than currently possible. Early experiments show potential for 10-100x improvements in certain financial calculations, with corresponding impacts on trading and risk management.
Explainable AI for investment will address the "black box" problem that has limited adoption of some AI approaches in regulated financial contexts. Solutions that provide clear reasoning for their recommendations will command premium prices.
Decentralized finance (DeFi) intelligence tools that help navigate the growing complexity of blockchain-based financial systems will see increasing demand. These platforms will help users identify opportunities and risks in this rapidly evolving space.
Behavioral finance AI that incorporates psychological insights into financial models will improve prediction accuracy. These systems will better anticipate market movements driven by human behavior rather than pure economic rationality.
Climate finance AI that helps assess and manage climate-related financial risks will become increasingly important as environmental factors impact investment decisions. These specialized tools will command premium prices due to the growing urgency of climate considerations.
Strategic Considerations for Businesses
For organizations looking to capitalize on this intersection, several strategic considerations should guide their approach:
- Focus on explainability and transparency, as financial decisions often require clear justification, particularly in regulated contexts.
- Develop robust security and privacy capabilities, as financial data is both sensitive and valuable.
- Build domain expertise in specific financial sectors or use cases rather than pursuing generic solutions.
- Address ethical considerations proactively, particularly regarding algorithmic bias in lending or investment decisions.
- Create clear methodologies for measuring and demonstrating ROI, as financial institutions typically require strong evidence of value.
The intersection of AI and finance represents one of the most established and profitable opportunities in the AI landscape. As financial institutions continue to seek competitive advantages and efficiency improvements, the demand for sophisticated financial AI will continue to grow, creating enduring opportunities for businesses that can effectively bridge the gap between financial expertise and AI capabilities.
Cybersecurity and AI: Protecting Digital Assets
The integration of AI into cybersecurity represents one of the most critical and rapidly growing intersections in the technology landscape. As cyber threats become increasingly sophisticated, AI-powered security solutions have emerged as essential tools in the ongoing battle to protect digital assets and infrastructure.
Market Size and Accelerating Growth
The global AI in cybersecurity market is experiencing explosive expansion. According to Grand View Research, the market size was valued at $14.9 billion in 2023 and is projected to reach $133.8 billion by 2030, growing at a remarkable CAGR of 36.8% from 2024 to 2030 [18]. This exceptional growth reflects the urgent need for more advanced security solutions to counter evolving threats.
What makes this intersection particularly valuable is the asymmetric nature of cybersecurity challenges, where defenders must protect against countless potential attack vectors. As one security expert notes, "AI in cybersecurity isn't just about automation—it's about fundamentally changing the economics of defense by enabling security teams to scale their capabilities against increasingly sophisticated adversaries."
Why Cybersecurity AI Creates Exceptional Value
Several factors make cybersecurity a particularly profitable AI-human intersection:
Persistent skills shortage: The global cybersecurity workforce gap exceeds 3.5 million positions, creating strong demand for AI solutions that can amplify the capabilities of existing security teams. Organizations are willing to pay premium prices for technologies that help address this critical shortage.
Evolving threat landscape: Cyber threats are continuously evolving in sophistication and scale, requiring equally advanced defensive capabilities. AI systems that can adapt to new attack patterns create significant value through improved protection.
High cost of breaches: The average cost of a data breach has reached $4.45 million in 2023, according to IBM's Cost of a Data Breach Report [19]. Solutions that effectively reduce breach risk command premium prices due to their direct impact on risk mitigation.
Regulatory pressure: Growing regulatory requirements around data protection and breach disclosure create strong incentives for organizations to invest in advanced security solutions. AI tools that improve compliance posture address a critical business need.
Profitable Business Models in Cybersecurity AI
Several business models have proven particularly successful in the cybersecurity AI space:
Threat detection and response platforms: AI-powered systems that identify and respond to security threats in real-time are seeing rapid adoption. Leading platforms report 60-80% faster threat detection compared to traditional approaches, with some reducing response times from hours to minutes or seconds.
Vulnerability management solutions: AI tools that continuously scan for and prioritize security vulnerabilities address a critical pain point for security teams. These solutions can reduce the vulnerability remediation backlog by 30-50% while focusing efforts on the most critical issues.
Security operations automation: AI systems that automate routine security tasks and investigations dramatically improve operational efficiency. Some solutions report enabling security teams to handle 3-5x more alerts with the same headcount.
Fraud detection systems: AI-powered fraud prevention tools that identify suspicious patterns in financial transactions or user behaviors command premium prices. These platforms can reduce fraud losses by 40-60% while minimizing false positives that impact legitimate users.
Predictive security analytics: Platforms that forecast potential security issues before they manifest enable proactive defense. These solutions can reduce security incidents by 20-30% through early intervention.
Real-World Success Stories
The theoretical potential of this intersection is being realized through numerous success stories:
Darktrace's Enterprise Immune System leverages advanced AI to detect, respond to, and neutralize cyber threats autonomously [20]. Their Antigena solution continuously analyzes the behavior of every device and user on the network to establish a baseline of normal activity and detect deviations. The implementation effectively thwarted ransomware attacks and insider threats, reduced response time from hours/days to seconds/minutes, and autonomously responded to abnormal behaviors before escalation.
CrowdStrike's Falcon Platform addresses the challenge of protecting countless endpoints from advanced threats by continuously monitoring and analyzing billions of events across global endpoints in real-time. Their AI technology detects patterns and anomalies indicating potential threats, offering a proactive rather than reactive approach to cybersecurity.
AI-Powered Threat Detection systems analyze network traffic patterns to identify potential threats, detecting subtle anomalies that might indicate a breach attempt. These systems can identify threats that traditional signature-based approaches would miss entirely.
Malware and Phishing Detection tools use AI systems trained to recognize characteristics of malicious software and fraudulent communications. The result is faster and more accurate identification of threats compared to traditional methods, with some solutions reducing successful phishing attacks by 60-70%.
Automated Incident Response platforms use AI-powered systems to automatically contain and mitigate threats once detected. This automation reduces response time and minimizes damage from security incidents, with some organizations reporting a 90% reduction in breach impact when using these tools.
Future Trends and Opportunities
Looking ahead, several trends are likely to further increase the value of this intersection:
Adversarial AI will become increasingly important as attackers deploy AI systems of their own. Defensive solutions that can counter AI-powered attacks will command premium prices due to the growing sophistication of these threats.
Zero-trust architecture enhanced by AI will transform security approaches, moving from perimeter defense to continuous verification. AI systems that can implement zero-trust principles without degrading user experience will see strong demand.
Supply chain security powered by AI will address the growing risks in software and hardware supply chains. Solutions that can verify the integrity of components and dependencies will become essential as supply chain attacks increase.
Quantum-resistant security enhanced by AI will prepare organizations for the post-quantum cryptography era. Tools that help identify and remediate quantum vulnerabilities will become increasingly valuable as quantum computing advances.
Human-AI teaming in security operations will evolve beyond simple automation to true collaboration. Platforms that effectively combine human judgment with AI capabilities will outperform either approach alone.
Strategic Considerations for Businesses
For organizations looking to capitalize on this intersection, several strategic considerations should guide their approach:
- Focus on reducing false positives, as alert fatigue remains a critical challenge for security teams. Solutions that deliver high precision create disproportionate value.
- Develop explainable AI that can articulate the reasoning behind security alerts, enabling human analysts to quickly understand and act on AI-generated insights.
- Build adaptive capabilities that can respond to evolving threats without requiring constant retraining or rule updates.
- Address privacy concerns proactively, as security monitoring often involves sensitive data. Privacy-preserving AI techniques will become increasingly important.
- Create clear metrics and ROI models that demonstrate security value, helping overcome the traditional challenge of quantifying security benefits.
The intersection of AI and cybersecurity represents one of the most urgent and substantial profit opportunities in the technology landscape. As digital threats continue to evolve in sophistication and impact, the demand for effective security AI will continue to grow, creating enduring opportunities for businesses that can effectively bridge the gap between security expertise and AI capabilities.
AI-based Marketing and Advertising: Personalized Persuasion
The integration of AI into marketing and advertising represents one of the most transformative and profitable intersections in the business landscape. By enabling unprecedented levels of personalization, optimization, and automation, AI is fundamentally changing how brands connect with customers and maximize their return on marketing investment.
Market Size and Explosive Growth
The global AI in marketing market is experiencing remarkable expansion. According to Grand View Research, the market size was valued at $15.9 billion in 2023 and is projected to reach $107.5 billion by 2030, growing at a CAGR of 31.5% from 2024 to 2030 [21]. This exceptional growth reflects marketers' recognition of AI's potential to dramatically improve campaign performance and customer engagement.
What makes this intersection particularly valuable is its direct impact on revenue generation and customer acquisition. As one marketing technology expert notes, "AI in marketing isn't just about efficiency—it's about fundamentally improving the economics of customer acquisition and retention through more precise targeting and personalization."
Why Marketing AI Creates Exceptional Value
Several factors make marketing and advertising a particularly profitable AI-human intersection:
Data-driven optimization: Marketing has always been a metrics-focused discipline, making it ideal for AI enhancement. AI systems can process vastly more campaign data than human analysts, identifying patterns and opportunities for improvement that would otherwise be missed.
Personalization imperative: Modern consumers increasingly expect personalized experiences, which are impossible to deliver at scale without AI. Solutions that enable effective personalization create significant competitive advantages.
Creative enhancement: AI tools that augment human creativity in content creation and campaign design address a critical bottleneck in marketing operations. These solutions can dramatically increase creative output while maintaining quality.
Channel complexity: The proliferation of marketing channels creates coordination challenges that AI can help solve. Tools that optimize cross-channel campaigns create substantial value through improved integration.
Profitable Business Models in Marketing AI
Several business models have proven particularly successful in the marketing AI space:
Predictive analytics platforms: AI-powered systems that forecast customer behavior and campaign performance are seeing rapid adoption. Leading platforms report 30-50% improvements in campaign ROI through more precise targeting and timing.
Content generation and optimization: AI tools that create, enhance, or personalize marketing content at scale address a critical pain point for marketers. These solutions can reduce content production costs by 40-70% while increasing output.
Customer journey orchestration: AI systems that coordinate marketing touchpoints across channels and stages create more coherent customer experiences. Some solutions report increasing conversion rates by 15-30% through improved journey management.
Programmatic advertising optimization: AI-enhanced platforms that automate ad buying and placement decisions are transforming media spending. These systems can improve advertising performance by 20-40% compared to traditional approaches.
Conversational marketing: AI-powered chatbots and virtual assistants that engage customers in personalized conversations are creating new engagement opportunities. Leading solutions report 30-50% increases in qualified leads through intelligent conversation management.
Real-World Success Stories
The theoretical potential of this intersection is being realized through numerous success stories:
Meta's AI Sandbox for Advertisers is testing AI-generated ads for Facebook with capabilities including text variations generation, text-to-image generation, and image outcropping to fit different aspect ratios [22]. This innovation positions Meta to have the best AI-driven mobile advertising platform, saving advertisers time in creating new image creatives and encouraging continuous process innovation.
Coca-Cola's "Create Real Magic" Contest showcased their partnership with Bain & Company and OpenAI to integrate AI into their systems. The campaign invited users to combine ChatGPT, DALL-E, and historic Coca-Cola ad creatives to create new works of art, demonstrating how brands can stay on top of market innovations while increasing customer engagement and brand awareness.
Calm App's Amazon Personalize Integration addressed the challenge of users spending too much time browsing content by implementing personalized content recommendations. Their dynamic rule recommends popular content in the user's preferred style while removing already-consumed content, resulting in a 3.4% increase in daily app use and demonstrating how AI can improve user experience through personalization.
Nike's AI-Generated Ad with Serena Williams created the "Never done evolving" campaign using AI to generate a match between Serena's younger self (1999) and modern self (2017). The 8-minute video ad was livestreamed on YouTube to 1.69 million subscribers, showcasing how brands can use historical data to create emotional connections with their audience.
ClickUp's AI-Powered Content Optimization used SurferSEO to optimize content while increasing both quality and quantity. Their implementation provided granular insights during content planning, created thorough content briefs, and monitored performance with data-backed insights. The results were impressive: over 150 articles published and 85% growth in non-branded organic traffic in 12 months.
Sephora's Virtual Assistant uses AI to provide personalized beauty recommendations, enhancing customer engagement and increasing conversion rates. This implementation demonstrates how AI can create more meaningful interactions with customers while driving business results.
McDonald's Mass Personalization leverages AI-driven personalized marketing campaigns to improve customer targeting and increase sales. Their approach shows how even large-scale businesses can create individualized experiences that resonate with customers.
Future Trends and Opportunities
Looking ahead, several trends are likely to further increase the value of this intersection:
Emotion AI that can detect and respond to customer emotional states will enable more nuanced marketing approaches. Early implementations show 20-40% improvements in campaign effectiveness through emotionally intelligent messaging.
Augmented creativity tools that serve as collaborative partners for marketers will transform content creation. These systems will suggest novel concepts and approaches that human marketers might not consider.
Predictive customer lifetime value models will enable more sophisticated acquisition and retention strategies. These approaches can increase marketing ROI by 30-50% through more precise resource allocation.
Privacy-preserving personalization will become increasingly important as data regulations tighten. Solutions that can deliver personalized experiences without compromising privacy will command premium prices.
Real-time experience optimization that continuously adjusts customer interactions based on immediate feedback will create more adaptive marketing systems. These approaches can improve conversion rates by 15-25% through dynamic optimization.
Strategic Considerations for Businesses
For organizations looking to capitalize on this intersection, several strategic considerations should guide their approach:
- Focus on augmenting rather than replacing human marketers, creating tools that enhance creativity and strategic thinking while automating routine tasks.
- Develop clear approaches to data ethics and privacy that go beyond compliance to build customer trust.
- Build capabilities that bridge marketing expertise with AI technical knowledge to ensure solutions address genuine marketing needs.
- Create clear methodologies for measuring AI marketing impact, helping overcome the attribution challenges that often complicate marketing technology evaluation.
- Invest in continuous learning capabilities that allow marketing AI to adapt to changing consumer behaviors and preferences.
The intersection of AI and marketing represents one of the most immediate and substantial profit opportunities in the business technology landscape. As organizations increasingly recognize the competitive necessity of AI-enhanced marketing, the demand for effective solutions will continue to grow, creating enduring opportunities for businesses that can effectively bridge the gap between marketing expertise and AI capabilities.
Conclusion: Navigating the AI-Human Profit Landscape
As we've explored throughout this article, the intersections of AI and human expertise offer unprecedented opportunities for profit and innovation across diverse industries. These nine key areas—content creation, education, business integration, legal services, healthcare, creative content, finance, cybersecurity, and marketing—represent the most promising territories for organizations and entrepreneurs seeking to capitalize on the AI revolution.
Key Insights and Patterns
Several important patterns emerge from our analysis of these profitable intersections:
Value creation through augmentation, not replacement: The most successful AI implementations enhance human capabilities rather than simply automating existing processes. The highest returns come from solutions that combine AI's computational power with human judgment, creativity, and domain expertise.
Industry-specific solutions outperform general approaches: Across all nine intersections, we see that AI solutions tailored to specific industry needs consistently command premium prices and achieve higher adoption rates. Deep domain knowledge remains essential for creating truly valuable AI implementations.
Data advantages create sustainable competitive moats: Organizations with unique, high-quality data assets are particularly well-positioned to create valuable AI solutions. The combination of proprietary data with AI capabilities creates defensible competitive advantages that are difficult to replicate.
Human-AI collaboration requires thoughtful design: Successful implementations carefully consider how humans and AI systems interact, creating interfaces and workflows that leverage the strengths of both. This design thinking is often what separates successful AI initiatives from failed ones.
Ethical considerations are becoming competitive factors: As AI adoption accelerates, organizations that proactively address ethical concerns around bias, privacy, and transparency are gaining advantages in talent acquisition, customer trust, and regulatory readiness.
Strategic Recommendations for Organizations
For businesses and entrepreneurs looking to capitalize on these profitable intersections, several strategic recommendations emerge:
- Identify your unique data and expertise advantages: Assess where your organization has distinctive data assets or domain expertise that could form the foundation of valuable AI solutions.
- Focus on augmentation over automation: Prioritize AI initiatives that enhance human capabilities rather than simply replacing human labor with automated processes.
- Build multidisciplinary teams: Successful AI implementation requires collaboration between domain experts, data scientists, engineers, designers, and business strategists. Cultivate these diverse skill sets within your organization.
- Develop clear AI ethics frameworks: Establish principles and processes for addressing ethical considerations in AI development and deployment before they become critical issues.
- Invest in AI literacy across your organization: Ensure that leaders and team members throughout your organization understand AI's capabilities, limitations, and strategic implications.
The Future Outlook
Looking ahead, we can expect these profitable intersections to continue evolving rapidly. Several trends will likely shape the landscape in the coming years:
Increasing democratization of AI capabilities: As AI tools become more accessible to non-technical users, the barriers to entry for creating AI-enhanced products and services will continue to fall.
Growing importance of explainable AI: As AI is applied to increasingly consequential decisions, the ability to explain and justify AI-generated recommendations will become a critical requirement.
Regulatory frameworks catching up to innovation: Expect increased regulatory attention to AI applications, particularly in high-stakes domains like healthcare, finance, and legal services.
New intersections emerging: As AI capabilities continue to advance, new profitable intersections will emerge that we cannot yet fully anticipate.
The organizations that will thrive in this evolving landscape will be those that remain adaptable, ethically grounded, and focused on creating genuine value through thoughtful integration of AI and human capabilities. The greatest opportunities lie not in pursuing AI for its own sake, but in identifying specific problems where the combination of AI and human expertise can create transformative solutions.
By focusing on these nine key intersections and applying the strategic principles we've outlined, organizations of all sizes can position themselves to capture significant value from the ongoing AI revolution. The future belongs not to AI alone, nor to human expertise in isolation, but to those who master the art of bringing them together in ways that create maximum value for all stakeholders.
Frequently Asked Questions
Which AI-human intersection offers the highest ROI currently?
While all nine intersections offer significant profit potential, healthcare and finance currently show the highest ROI for AI implementations. Healthcare AI solutions addressing diagnostic accuracy and operational efficiency can deliver ROI of 200-400% within 12-24 months of implementation. Similarly, financial AI applications in areas like algorithmic trading and risk assessment often show returns of 150-300% within the first year.
However, the "best" intersection varies significantly based on your organization's existing expertise, data assets, and market position. Companies should prioritize areas where they have domain knowledge and unique data advantages rather than simply pursuing the highest general ROI figures.
How can small businesses benefit from AI-human intersections?
Small businesses can effectively capitalize on AI-human intersections through several approaches:
- Leverage AI platforms and APIs: Rather than building AI capabilities from scratch, small businesses can use existing platforms and APIs from providers like OpenAI, Google, and Microsoft to add AI functionality to their products and services.
- Focus on niche applications: Target specific industry problems where your domain expertise gives you an advantage in creating tailored AI solutions.
- Start with process optimization: Implement AI tools that improve internal efficiency before moving to customer-facing applications. This approach typically offers quicker returns with lower risk.
- Consider AI-as-a-service models: Subscription-based AI tools can provide sophisticated capabilities without requiring large upfront investments in technology or talent.
- Build strategic partnerships: Collaborate with AI technology providers or complementary businesses to create joint solutions that leverage each partner's strengths.
The democratization of AI tools means that even small organizations can now implement powerful AI capabilities that would have been inaccessible just a few years ago.
What skills should professionals develop to capitalize on these opportunities?
Professionals looking to thrive at AI-human intersections should develop a combination of technical, domain, and translational skills:
Technical skills provide the foundation for understanding AI capabilities and limitations:
- Basic data literacy and statistical thinking
- Fundamental AI/ML concepts and terminology
- Prompt engineering for generative AI systems
- Ability to evaluate AI solution quality and performance
Domain expertise remains essential for creating valuable AI applications:
- Deep understanding of industry-specific processes and challenges
- Knowledge of relevant regulations and compliance requirements
- Awareness of industry data sources and their limitations
Translational skills bridge the gap between technical and business considerations:
- Ability to identify high-value use cases for AI implementation
- Skills in redesigning workflows to effectively incorporate AI
- Change management capabilities to drive AI adoption
- Ethical reasoning about AI applications and implications
The most valuable professionals will be those who can serve as "bridges" between technical AI capabilities and practical business applications, translating between these different domains.
How quickly can businesses expect to see returns from AI investments?
The timeline for returns on AI investments varies significantly based on the type of application and implementation approach:
Quick wins (3-6 months):
- Process automation applications
- AI-enhanced analytics dashboards
- Basic chatbots and virtual assistants
- Content optimization tools
Medium-term returns (6-18 months):
- Predictive maintenance systems
- Customer segmentation and personalization
- Fraud detection and risk assessment
- Document processing and analysis
Longer-term investments (18+ months):
- Comprehensive AI transformation programs
- Advanced R&D applications
- Complex diagnostic systems requiring regulatory approval
- Fundamental business model innovations
Organizations should typically pursue a portfolio approach, balancing quick wins that demonstrate value and build momentum with longer-term strategic investments that may offer greater competitive advantages.
What are the main challenges in implementing AI in these industries?
Organizations face several common challenges when implementing AI across the intersections we've discussed:
Data quality and accessibility issues: Many AI initiatives struggle with insufficient, fragmented, or poor-quality data. Successful implementation requires investing in data infrastructure and governance before or alongside AI development.
Integration with existing systems: AI solutions must work within complex technology ecosystems. Challenges in API development, legacy system integration, and workflow redesign often delay or diminish returns.
Talent and expertise gaps: Despite growing AI education programs, demand for AI talent still far exceeds supply. Organizations struggle to recruit, retain, and effectively deploy AI specialists.
Change management and adoption: Technical implementation is often easier than driving organizational adoption. Resistance to change, concerns about job displacement, and lack of AI literacy among stakeholders can undermine otherwise promising initiatives.
Ethical and regulatory considerations: Navigating evolving regulations, addressing bias and fairness concerns, and ensuring appropriate transparency and explainability add complexity to AI implementations.
Scaling beyond pilots: Many organizations successfully implement AI pilots but struggle to scale these successes across the enterprise. Moving from proof-of-concept to production requires different approaches and capabilities.
Addressing these challenges requires a holistic approach that considers technology, people, processes, and governance rather than treating AI implementation as a purely technical exercise.
How is AI changing the nature of work in these industries?
Across all nine intersections, AI is reshaping work in several consistent patterns:
Automation of routine tasks: Repetitive, rule-based activities are increasingly being automated, freeing human workers to focus on more complex and creative aspects of their roles.
Augmentation of human capabilities: AI tools are enhancing human decision-making by providing insights, recommendations, and analysis that would be difficult or impossible for humans alone.
Creation of new specialist roles: New positions are emerging that focus on AI implementation, oversight, and optimization, such as prompt engineers, AI ethicists, and human-AI collaboration designers.
Increased emphasis on uniquely human skills: As AI handles more routine cognitive tasks, skills like creativity, emotional intelligence, ethical reasoning, and complex problem-solving are becoming more valuable.
Evolution of management practices: Supervising teams that include both human and AI components requires new management approaches and performance metrics.
Rather than wholesale replacement of jobs, we're seeing a gradual evolution where job roles are being redefined around the complementary strengths of humans and AI. The most successful organizations are those that proactively manage this transition, helping employees adapt to changing skill requirements and work processes.
We also suggest checking out our materials on other AI and earning-related topics; here are our best articles:
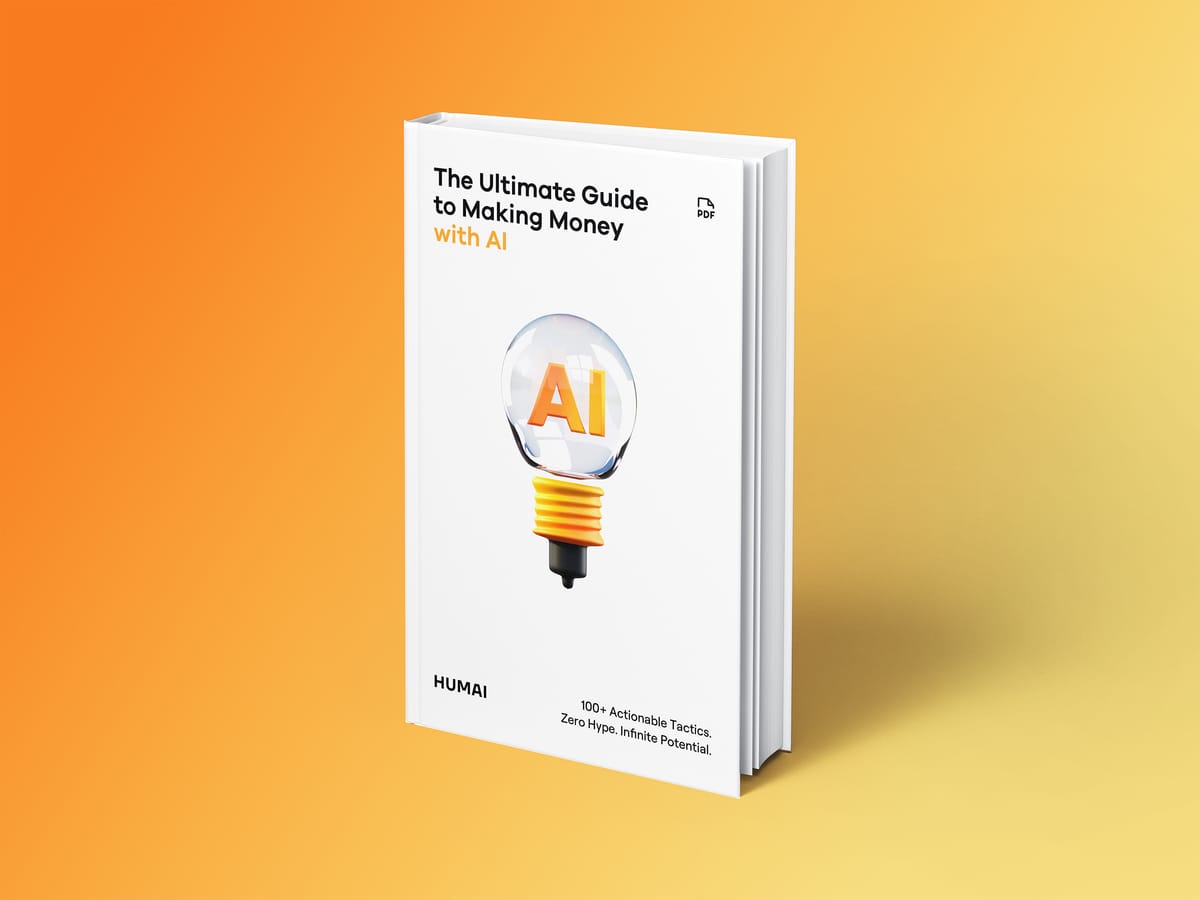
PDF Book
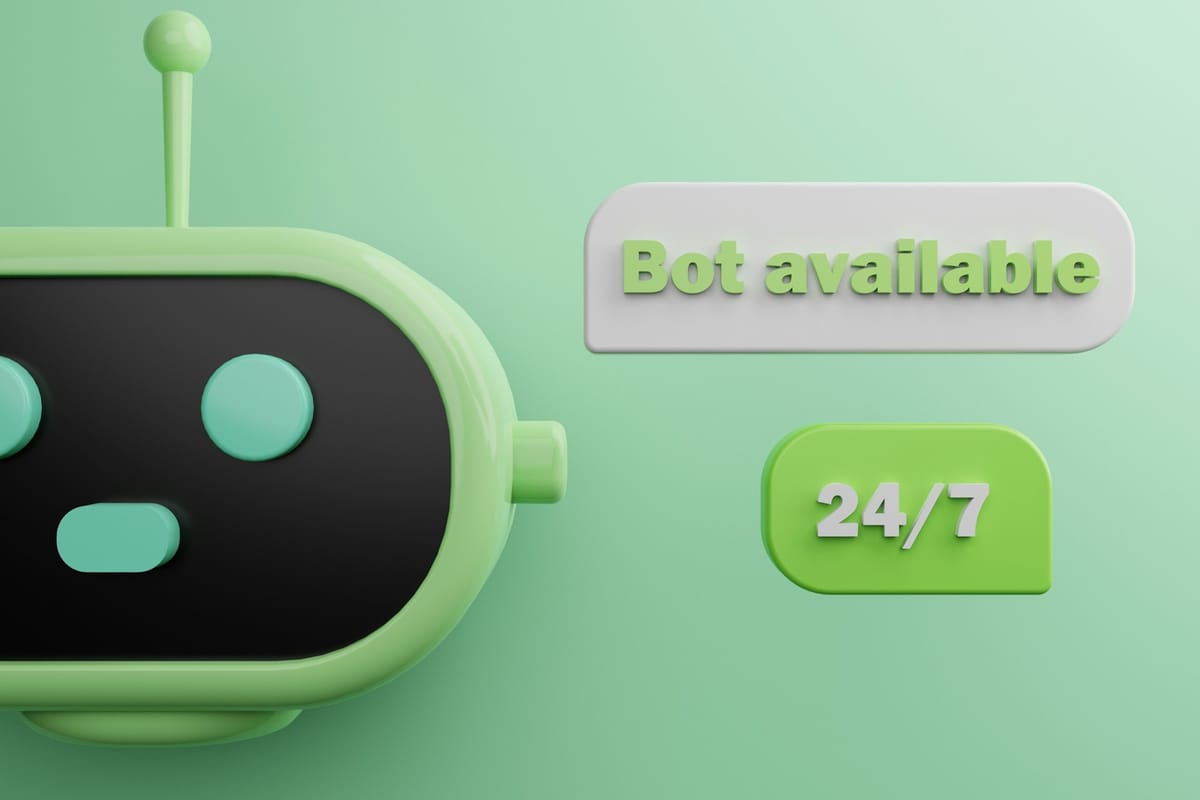
Deep Guide
References
[1] Precedence Research. (2023). Artificial Intelligence Market Size to Surpass US$ 1,811.8 Bn by 2030. Retrieved from https://www.precedenceresearch.com/artificial-intelligence-market
[2] MarketsandMarkets. (2023). AI Content Creation Market - Global Forecast to 2026. Retrieved from https://www.marketsandmarkets.com/
[3] Deloitte. (2024). AI and Content: The Human Element in Machine Learning. Deloitte Digital Insights.
[4] DataFeedWatch. (2025). 11 Best AI Advertising Examples of 2025. Retrieved from https://www.datafeedwatch.com/blog/best-ai-advertising-examples
[5] Netguru. (2025). AI Content Repurposing: Top Tools and Success Stories in 2025. Retrieved from https://www.netguru.com/blog/ai-content-repurposing-top-tools-and-success-stories-in-2025
[6] Grand View Research. (2024). Artificial Intelligence In Education Market Size Report, 2032. Retrieved from https://www.grandviewresearch.com/industry-analysis/artificial-intelligence-ai-education-market-report
[7] Coursera. (2024). Annual Impact Report. Coursera Inc.
[8] Precedence Research. (2023). Artificial Intelligence Market Size to Surpass US$ 1,811.8 Bn by 2030. Retrieved from https://www.precedenceresearch.com/artificial-intelligence-market
[9] Microsoft. (2025). How Real-World Businesses Are Transforming with AI. Retrieved from https://blogs.microsoft.com/blog/2025/04/22/https-blogs-microsoft-com-blog-2024-11-12-how-real-world-businesses-are-transforming-with-ai/
[10] Grand View Research. (2024). Legal AI Market Size Report, 2032. Retrieved from https://www.grandviewresearch.com/industry-analysis/legal-ai-market-report
[11] Legartis. (2025). Trends in AI Contract Analysis. Retrieved from https://www.legartis.ai/blog/trends-ai-contract-analysis
[12] Grand View Research. (2024). Artificial Intelligence In Healthcare Market Size Report, 2032. Retrieved from https://www.grandviewresearch.com/industry-analysis/artificial-intelligence-ai-healthcare-market
[13] Digital Defynd. (2025). AI in Healthcare Case Studies. Retrieved from https://digitaldefynd.com/IQ/ai-in-healthcare-case-studies/
[14] Grand View Research. (2024). Generative AI In Music Market Size Report, 2032. Retrieved from https://www.grandviewresearch.com/industry-analysis/generative-ai-in-music-market-report
[15] Christie's. (2018). Is Artificial Intelligence Set to Become Art's Next Medium? Retrieved from https://www.christies.com/features/A-collaboration-between-two-artists-one-human-one-a-machine-9332-1.aspx
[16] MarketsandMarkets. (2024). AI in Finance Market - Global Forecast to 2028. Retrieved from https://www.marketsandmarkets.com/Market-Reports/ai-in-finance-market-90552286.html
[17] Digital Defynd. (2025). AI in Banking Case Studies. Retrieved from https://digitaldefynd.com/IQ/ai-in-banking-case-studies/
[18] Grand View Research. (2023). Artificial Intelligence In Cybersecurity Market Size Report, 2030. Retrieved from https://www.grandviewresearch.com/industry-analysis/artificial-intelligence-cybersecurity-market-report
[19] IBM. (2023). Cost of a Data Breach Report. IBM Security.
[20] Digital Defynd. (2025). Agentic AI in Cybersecurity Case Studies. Retrieved from https://digitaldefynd.com/IQ/agentic-ai-in-cybersecurity-case-studies/
[21] Grand View Research. (2023). Artificial Intelligence In Marketing Market Size Report, 2030. Retrieved from https://www.grandviewresearch.com/industry-analysis/artificial-intelligence-marketing-market-report
[22] DataFeedWatch. (2025). 11 Best AI Advertising Examples of 2025. Retrieved from https://www.datafeedwatch.com/blog/best-ai-advertising-examples