Progress notes are the lifeblood of patient care. These structured entries in a medical record track a patient’s clinical status over time, serve as communication tools between providers, and act as legal documents. But for decades, progress notes have been a source of stress for clinicians—time-consuming to create, prone to inconsistencies, and often lacking in standardization. With the explosion of artificial intelligence (AI), particularly in the natural language processing (NLP) space, there’s a tectonic shift underway in how these notes are generated, reviewed, and utilized. AI is poised to not only streamline the documentation process but also elevate the quality of care.
In this article, we explore the evolution of progress notes, the critical role AI plays in their modernization, and the implications for healthcare professionals, designers of health tech systems, and patients alike.
Understanding the Problem with Traditional Progress Notes
The traditional method of generating progress notes often involves clinicians manually documenting encounters in electronic health records (EHRs). While digital systems replaced paper records, they didn't fundamentally change the workflow. Clinicians still need to chart detailed narratives of each encounter—sometimes after long shifts or during brief administrative gaps.
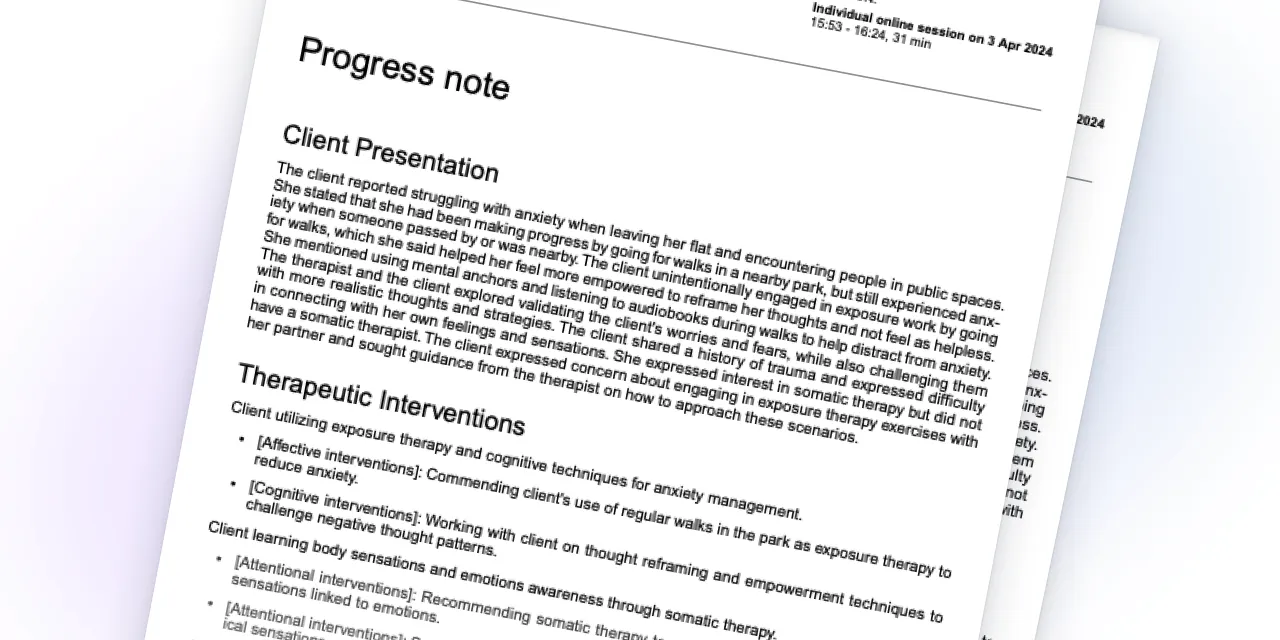
This process is riddled with challenges:
- Time Consumption: On average, clinicians spend nearly half of their working hours on documentation.
- Burnout: The cognitive load of keeping detailed notes on every patient encounter contributes to emotional exhaustion.
- Inconsistencies: Free-text entries vary widely in quality and completeness.
- Interoperability Issues: Notes often don’t follow standardized formats, complicating data exchange between systems.
These challenges collectively impact not just the efficiency of care delivery but also patient outcomes. Accurate, timely, and context-rich documentation is essential in avoiding medical errors, ensuring continuity of care, and meeting regulatory requirements.
Enter AI: A Catalyst for Smarter Progress Notes
AI, particularly NLP and machine learning (ML), has emerged as a powerful tool for automating and augmenting clinical documentation. Rather than replacing clinicians, AI supports them—capturing key insights, organizing them logically, and generating readable, compliant notes.
The most advanced systems today can transcribe spoken conversations in real time, recognize and extract medical terms, symptoms, and diagnoses, follow structured note templates such as SOAP (Subjective, Objective, Assessment, Plan), learn and adapt to individual providers’ documentation styles, and seamlessly integrate with electronic health records (EHRs) to auto-populate relevant patient data.The result is a radical improvement in both the efficiency and quality of progress notes.
AI-Powered Progress Notes in Practice
Several companies and health systems have already integrated AI tools for clinical note-taking with measurable benefits.
For instance, tools like Nuance Dragon Ambient eXperience (DAX) use ambient listening technology to passively capture patient-provider conversations, transcribe them, and convert them into structured progress notes. These drafts are then reviewed and signed off by the clinician, saving hours per week.
Startups like Suki and DeepScribe offer similar solutions using mobile apps and wearable devices, allowing physicians to document while on the move.
Hospital systems employing these technologies report:
- Up to 70% reduction in time spent on documentation.
- Higher clinician satisfaction and reduced burnout.
- Better note quality and completeness.
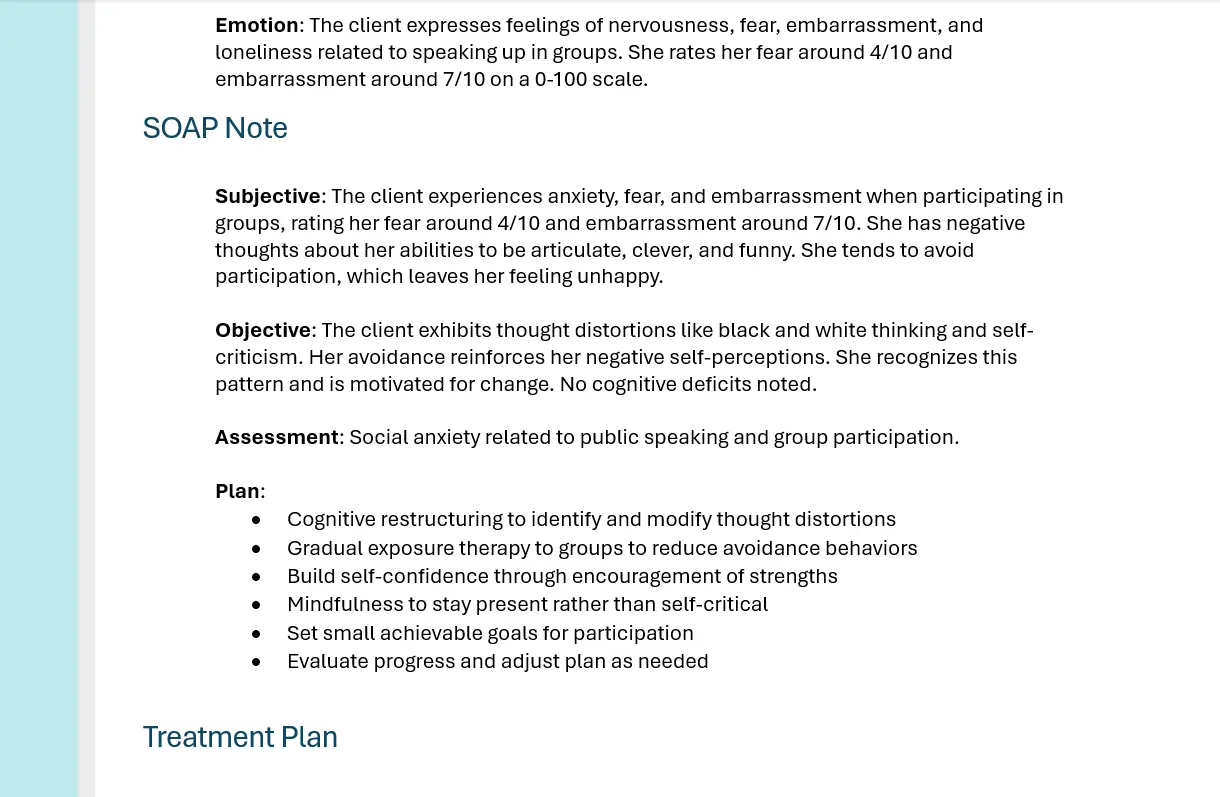
Beyond Transcription: Intelligent Understanding
AI in progress notes is not limited to voice recognition and transcription. The real game-changer is contextual understanding.
Modern AI models are trained not just to identify words but to comprehend the clinical context in which they're used. For example, if a patient says “I’ve been feeling tightness in my chest since yesterday,” a smart system will recognize this as a potential symptom of cardiac distress, flag it, and prompt further inquiry or escalation.
These models also factor in previous notes, test results, and medical history to avoid redundancies or contradictions. This contextual layer adds significant value, especially in chronic disease management or multi-specialty care environments.
Key AI Tools Powering Smarter Progress Notes
Several AI-powered tools stand out in the market today for their functionality and clinical impact. Each focuses on reducing documentation burdens while enhancing accuracy and usability for healthcare providers:
- Nuance DAX (Dragon Ambient eXperience)
Uses ambient listening to automatically capture and transcribe patient-provider conversations during clinical encounters. It then generates structured progress notes that align with SOAP or other medical documentation standards, ready for physician review and approval. - Suki
Acts as a voice-enabled digital assistant designed to reduce the time spent on documentation. Suki allows physicians to dictate notes naturally, while the AI handles formatting, medical term recognition, and integration into EHR systems. - DeepScribe
Offers real-time transcription and contextual note generation by listening in on patient visits. DeepScribe’s AI understands clinical language, organizes content into structured formats, and integrates directly into EHRs for seamless updates. - Abridge
Focuses on consumer-facing and clinician tools that summarize medical conversations. For healthcare professionals, it creates highlights and structured documentation from recorded visits, while ensuring transparency and patient-accessible summaries. - Amazon HealthScribe
A newer entrant that provides medical transcription services through AWS, designed to integrate directly into applications. It offers speaker separation, medical entity recognition, and summary generation—all through scalable cloud infrastructure.
These tools emphasize core functionalities such as real-time transcription, medical NLP, EHR integration, and intelligent formatting. Their shared goal is to turn documentation from a manual burden into an ambient, almost invisible part of the clinical experience.
Design Matters: UX and UI of AI-Driven Note Systems
The success of AI for progress notes also hinges on design. A poorly implemented AI system—no matter how powerful—can create friction and mistrust among users. Clinicians need transparency, control, and seamless integration into existing workflows.
Designers and developers must prioritize explainability—ensuring users understand what the AI is doing and why—alongside editability, allowing clinicians to easily revise or override AI-generated content. Equally important is minimizing disruption by creating interfaces that enhance rather than interrupt existing workflows, and maintaining strong data privacy standards with clear indicators of what information is being recorded, stored, or shared, particularly in compliance with regulations like HIPAA.
Good design ensures that AI doesn’t feel like an intrusive tool, but a natural extension of the clinical environment.
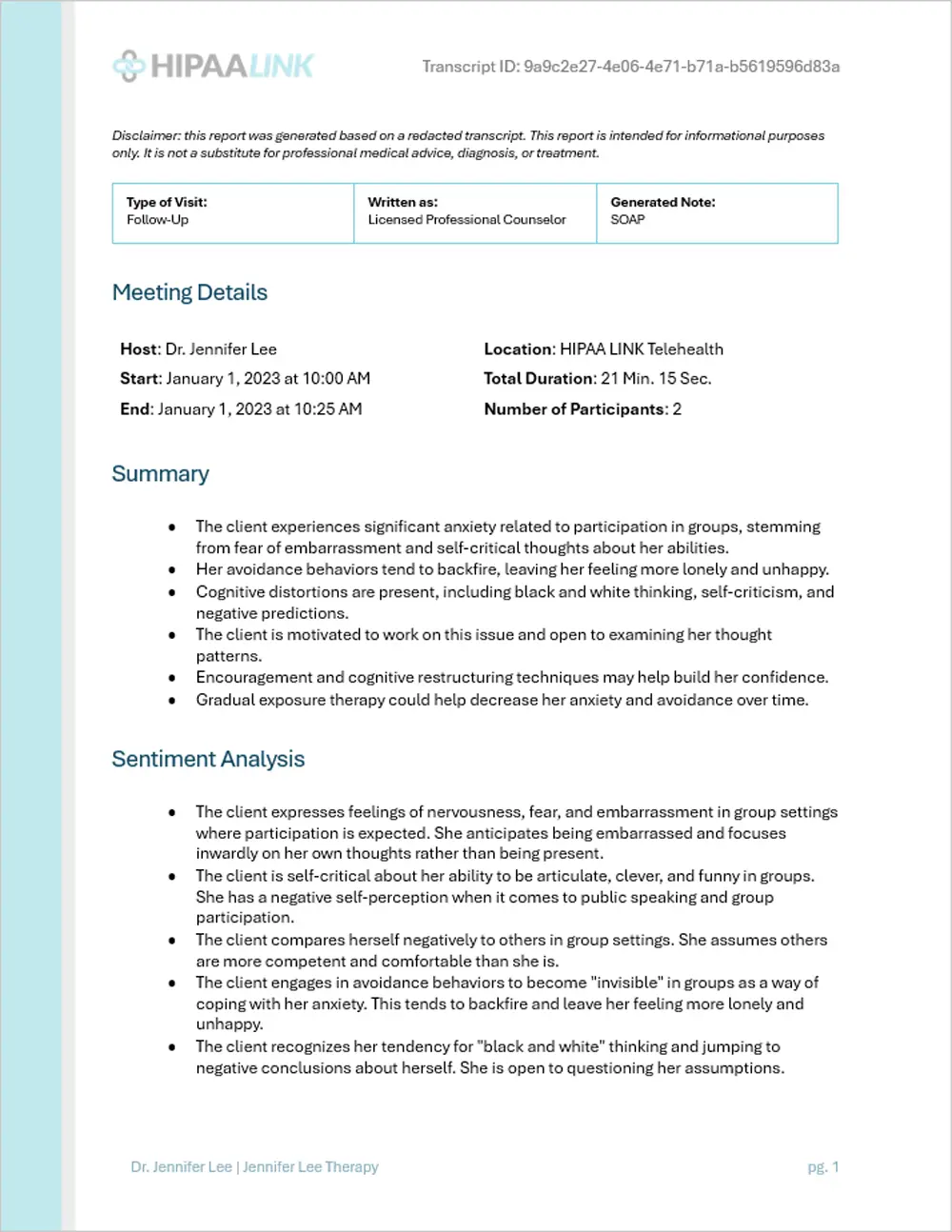
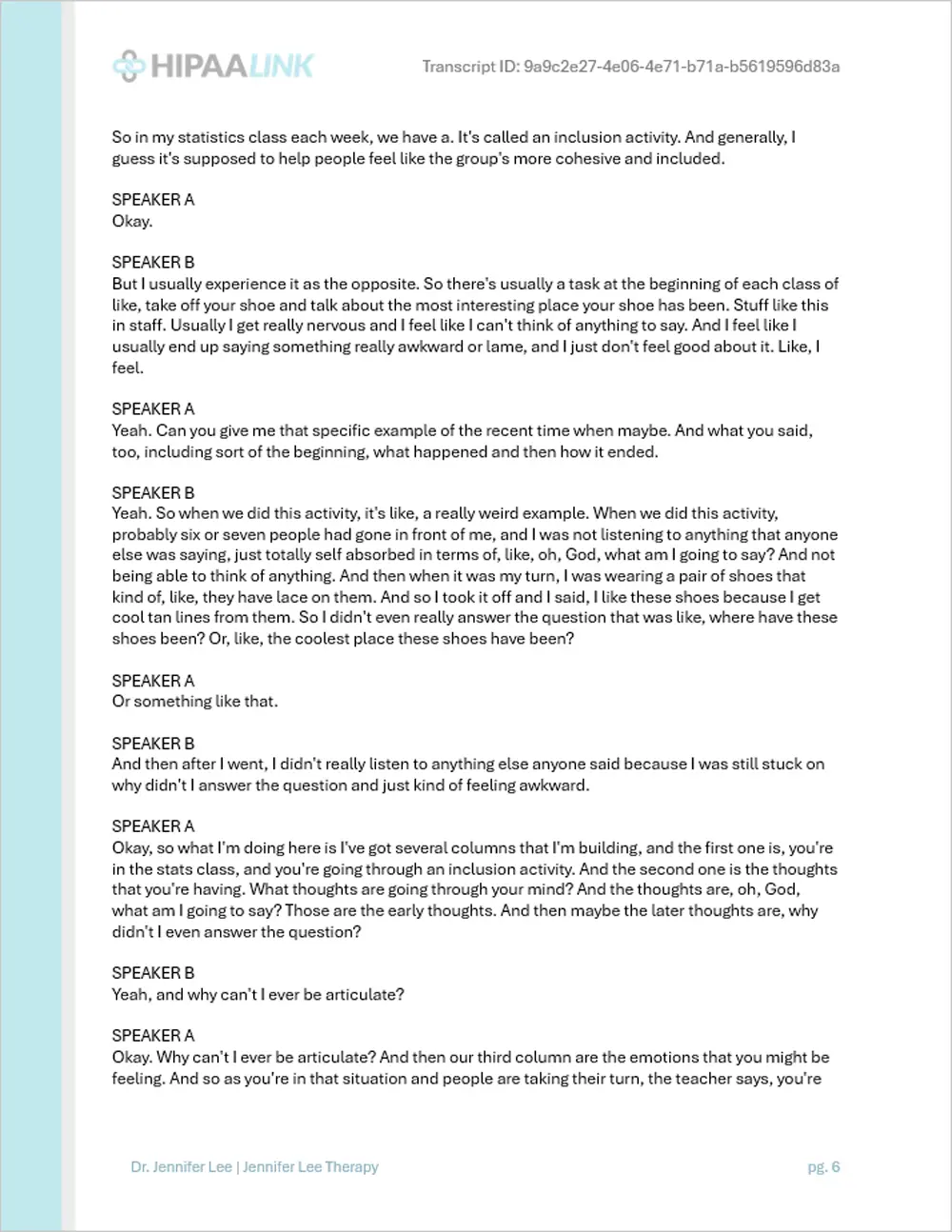
Ethical and Legal Considerations
Automating progress notes raises important questions around privacy, accountability, and bias.
If a mistake is made in an AI-generated note—say, a misinterpreted symptom or incorrect medication—who is responsible? The provider? The vendor? The hospital?
To mitigate these concerns, most systems are designed as assistive rather than autonomous. The clinician remains the final gatekeeper. Nonetheless, rigorous validation, audit trails, and continuous training are essential to ensure safety and trust.
Data privacy is another paramount concern. Conversations between patients and clinicians are sensitive, and recording them—especially with always-on ambient AI—requires clear patient consent, secure data handling, and strict access controls.
What the Future Holds
As models like GPT-4, Med-PaLM, and other large language models (LLMs) continue to improve, we can expect AI-generated progress notes to become even more nuanced, accurate, and proactive.
Future systems could:
- Summarize multi-specialty notes into unified patient narratives.
- Automatically flag inconsistencies or missing information.
- Generate predictive insights based on documented symptoms.
- Translate notes into layman's terms for patient access.
- Adapt to global healthcare systems with multilingual support.
In tandem with wearable health devices and patient-reported outcomes, AI could create a real-time, continuously updated health journal for every individual—a living progress note that evolves alongside the patient.
Challenges to Widespread Adoption
Despite its promise, AI in progress notes is not without friction. Several factors slow adoption:
- Cost: Implementing AI solutions can be expensive for smaller clinics.
- Training: Staff need time to learn and trust new systems.
- Regulatory Hurdles: Certification and compliance processes are still catching up with technology.
- Infrastructure: Many legacy EHR systems lack the APIs or flexibility to integrate cutting-edge AI.
Overcoming these hurdles requires not just technical innovation but also collaboration between stakeholders—healthcare providers, technologists, policymakers, and patients.
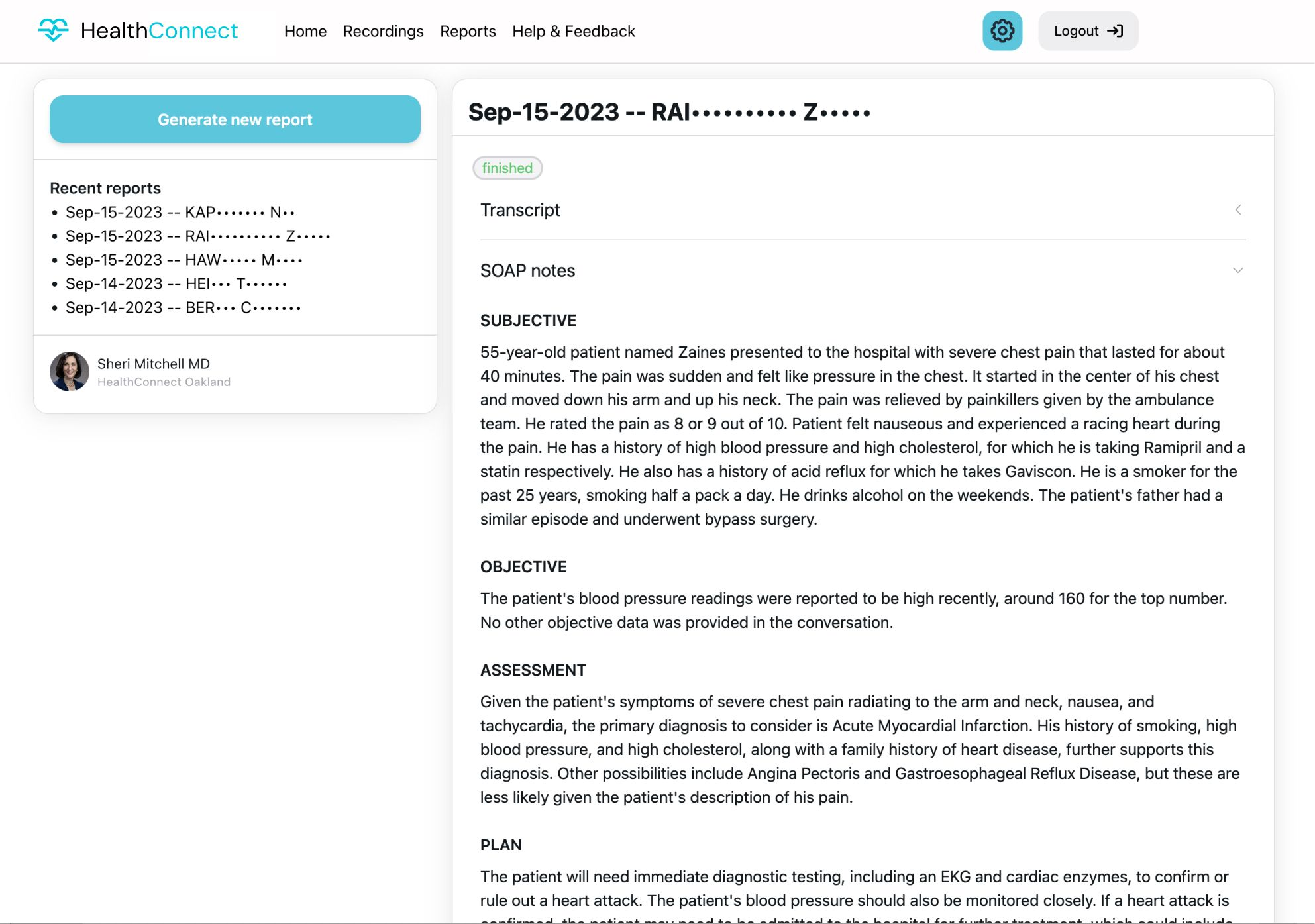
Real-world Use Cases: AI Clinical Documentation Success Stories
Real-world examples vividly illustrate AI's potential in clinical documentation. Healthcare providers using platforms such as Nuance DAX, Suki, and DeepScribe report substantial efficiency gains and improved clinician satisfaction. For instance, Nuance's ambient technology reduces documentation time by up to 70%, significantly mitigating clinician burnout and improving patient care quality.
Integrating AI with Existing Clinical Systems
Effective integration is crucial for the successful deployment of AI in clinical settings. Modern AI systems offer seamless compatibility with existing electronic health records (EHR) platforms, ensuring minimal disruption. APIs and standardized integration processes allow rapid onboarding, reducing the barrier to entry for hospitals and clinics of varying sizes and technological capacities.
Future Trends: AI's Role in Personalized Healthcare
The future of AI in clinical documentation extends beyond note-taking. Advanced predictive analytics powered by AI could soon allow clinicians to anticipate patient needs proactively. Integration with wearable technology and patient-generated data could further enhance personalized care delivery, fostering a more comprehensive and real-time health management system.
Whant to learn more about Clinical Notes AI? Read also What Are AI Clinical Notes and How Are They Changing Healthcare?
FAQ
How does AI improve clinical documentation accuracy?
AI leverages Natural Language Processing (NLP) and machine learning to accurately interpret medical terms, symptoms, and contexts, significantly reducing errors common in manual documentation.
What are the main barriers to adopting AI in clinical settings?
Primary barriers include cost, training requirements, regulatory hurdles, and compatibility issues with legacy electronic health record (EHR) systems.
Are AI-generated progress notes legally valid?
Yes, AI-generated notes are legally valid once reviewed and approved by a licensed clinician. Clinicians remain responsible for the accuracy and completeness of these documents.
How does AI impact clinician workload and burnout?
AI significantly reduces the time clinicians spend on documentation, directly addressing one of the major contributors to burnout by allowing more focus on patient care.
Can AI in clinical documentation compromise patient privacy?
Modern AI systems are designed with robust data security and privacy measures, ensuring compliance with regulations such as HIPAA. Patient consent and secure data handling practices are mandatory.
The Broader Implications
The impact of AI on progress notes extends far beyond documentation. It influences medical education, patient empowerment, and even population health analytics. With high-quality, standardized notes, data scientists can mine trends, researchers can study interventions, and administrators can spot inefficiencies. AI-driven documentation also improves continuity of care across geographies, providers, and time spans.
In a world increasingly defined by data, structured and intelligent documentation is foundational. AI is not just transforming how we write notes—it’s transforming how we understand and deliver care.
The Best Free AI Tools for Clinical and Medical Notes in 2025
Why AI-Powered Clinical Documentation Matters
Efficient, accurate documentation is the backbone of modern healthcare. Whether you're a physician, nurse, or medical student, AI-powered tools can help:
- Save time with automated note generation
- Reduce errors through structured templates
- Improve patient outcomes with consistent, clear records
The latest free AI tools in 2025 streamline everything from SOAP notes to discharge summaries while maintaining compliance and security standards.
Top Free AI Clinical Note Generators in 2025
1. AI Clinical Notes (Web-Based Solutions)
Advanced language models that generate professional medical records in seconds based on:
- Patient input
- Diagnoses
- Dictated observations
Specialized Options:
- AI nursing notes for bedside documentation, shift reports, and long-term care assessments
Key Features:
✔ Autocomplete for SOAP and progress notes
✔ Specialty-specific templates (pediatrics, psych, ER, etc.)
✔ HIPAA-compliant cloud storage
✔ PDF export capability
2. AI Progress Note Generator
Ideal for quick updates post-rounds with adaptive learning:
- Learns your documentation style over time
- Auto-generates concise, structured notes
Look For:
🔹 EHR integration (where available)
🔹 Customizable macros for repetitive entries
🔹 Structured subjective/objective data fields
3. AI Doctor's Note Generator
Creates compliant documentation in <60 seconds for:
- Return-to-work notes
- School absence notes
- Medical leave documentation
Standard Fields:
• Diagnosis/reason for absence
• Duration and activity restrictions
• Provider signature block
Security & Accessibility Features
Modern platforms offer:
- Secure clinical notes AI login with:
- Two-factor authentication
- Encrypted note storage
- Version history tracking
- Team functionality for multi-user access
- Cross-device synchronization
Advanced Functionality in 2025's Tools
Clinical Intelligence Capabilities
The best AI medical notes generators now:
- Recognize clinical terminology and ICD-10 codes
- Auto-suggest medications with dosage guidelines
- Incorporate patient history contextually
Template Libraries
Includes ready-to-use formats for:
Note Type | Use Case |
---|---|
SOAP | Subjective, Objective, Assessment, Plan |
DAP | Data, Assessment, Plan |
SBAR | Situation, Background, Assessment, Recommendation |
Export Options
All tools should provide:
- Patient notes PDF export with:
- Custom clinic branding
- Timestamp and author identification
- Field inclusion/exclusion options
- EHR-compatible file formats
Getting Started with AI Documentation
For New Users:
- Review provided clinical notes examples
- Start with template-based notes
- Gradually customize to your workflow
For Institutions:
- Verify HIPAA/GDPR compliance
- Train staff on AI-assisted documentation
- Monitor usage analytics for optimization
The Future of AI in Medical Documentation
Emerging trends to watch:
- Voice-to-note dictation with real-time formatting
- Automated coding suggestion engines
- Predictive text based on specialty-specific patterns
Note: Always verify generated notes for accuracy before finalizing patient records.