AI-powered clinical note applications are changing how doctors document patient visits — automating the note-taking process with intelligent tools. These platforms use cutting-edge speech recognition, natural language processing (NLP), and even generative AI to transcribe and summarize doctor-patient conversations into structured medical notes. The result? Clinicians spend less time typing and more time caring for patients, with fewer errors and more human interactions . In this popular-science overview, we’ll explore what Clinical Notes AI apps are, how they work, real-world use cases, leading solutions, benefits for providers and patients, current challenges (like privacy and accuracy), and future trends shaping this rapidly evolving field.
What Are Clinical Notes AI Apps?
Clinical Notes AI apps and platforms are software solutions that automatically generate or assist in creating medical documentation using artificial intelligence. In a typical doctor’s visit, instead of the physician typing or writing notes by hand after the appointment, an AI “listens” to the conversation (through a microphone or telehealth audio) and produces a written clinical note of the encounter . Think of it as a smart, invisible medical scribe: it transcribes what was said, understands the context and medical terminology, and organizes the information into the standard note format (often SOAP: Subjective, Objective, Assessment, Plan).
These AI platforms go beyond basic transcription. They employ advanced NLP and medical ontologies to recognize clinical concepts — for example, knowing “headache for two weeks” is a symptom in history, whereas “50 mg metoprolol daily” is a medication in the plan. Unlike a generic voice recorder, an AI clinical notes app can differentiate symptoms, diagnoses, medications, and other key details to build a coherent summary . In essence, the AI is trained in the “language of medicine,” allowing it to capture the full patient story in a structured, usable format.
Many such apps are cloud-based assistants that run on mobile devices or computers, integrating with electronic health record systems. They continuously improve by learning from large datasets of medical conversations and documentation. In 2025, some of the most advanced platforms even use large language models (LLMs) like GPT-4 (or domain-specific models) to enhance understanding and note generation . This means they don’t just transcribe words; they truly “comprehend” context and can draft well-formed clinical narratives almost like a human expert would.
Read also:
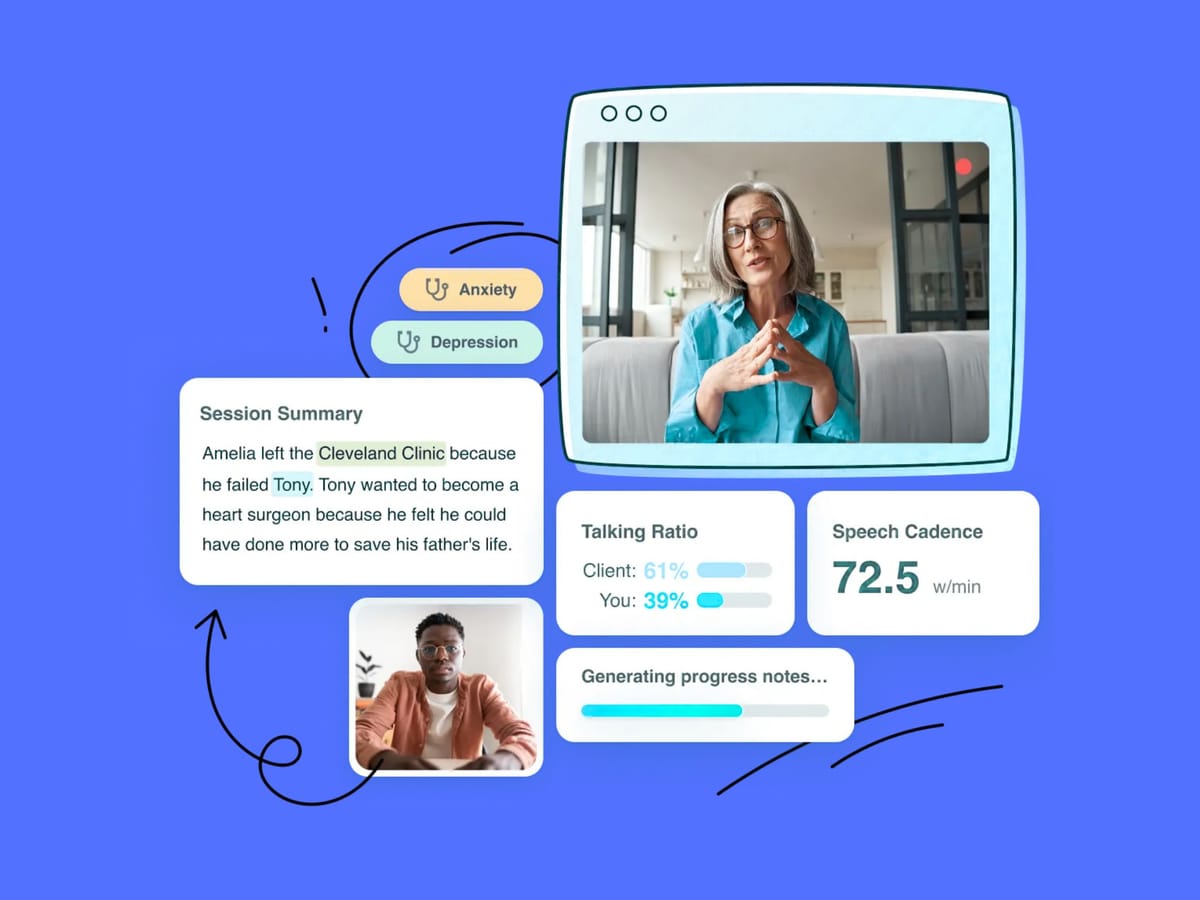
Who Are They For?
These AI note-taking solutions are designed for healthcare professionals who deal with documentation — primarily doctors, but also nurses, physician assistants, therapists, and other clinicians who write clinical notes. Any provider who spends a significant part of their day typing up patient encounters could benefit. Hospital administrators and clinic managers are also interested in such tools as a way to improve workflow efficiency and reduce burnout on their teams.
- Physicians in primary care: to lighten the load of routine visit notes and free up time for more patients or more personal attention during visits.
- Specialists and surgeons: to document complex discussions (e.g. oncology consultations, cardiology follow-ups) without interrupting the flow of conversation by stopping to type.
- Mental health professionals: to capture therapy session details, where nuance and exact phrasing can be important (some AI even picks up on tone or emotional cues in sessions) .
- Telemedicine providers: to generate real-time notes during virtual visits, where an AI can work in the background of a video call. This is especially useful for telehealth platforms aiming for quick turnaround in documentation .
- Healthcare IT and administrators: to integrate these tools into their clinics’ workflow, improving overall productivity. Leaders in healthcare business circles (see our AI Business section) are looking at these platforms as a way to scale services and cut costs by automating tedious processes.
Even smaller practices and rural clinics are adopting AI assistants to stay competitive and manage documentation with limited staff . In short, Clinical Notes AI apps are for anyone in healthcare who wants to spend less time on paperwork and more time on patients. (If improving efficiency is your goal, our Productivity guides show how AI can streamline many workflows, not just in medicine.)
The Documentation Burden: Why Automation is Needed
Modern healthcare is in the midst of an information overload. Studies show that U.S. clinicians spend nearly half their workday on documentation and administrative tasks rather than direct patient care . In practice, this means doctors often spend their evenings or weekends finalizing electronic health records (EHR) notes, leading to what’s grimly dubbed the “second shift” of paperwork. This imbalance not only eats into personal time but also contributes heavily to physician burnout . Inadequate time for charting and the stress of clunky EHR systems nearly triple the odds of burnout in physicians, according to research .
Beyond clinician well-being, there’s a patient safety aspect: when doctors are tired and rushed by documentation demands, errors can creep in and patient interactions suffer . It’s not uncommon to see a doctor pecking at a keyboard or navigating dropdown menus during an appointment, rather than making eye contact with the patient. Traditional methods (manual typing, dictation without context, or hiring human scribes) each have drawbacks in efficiency or cost.
This is the pain point that Clinical Notes AI platforms aim to solve. By automating note-taking, these tools address several issues:
- Time consumption: Instead of 10–15 minutes writing a note, an AI-generated note might require just a quick review or edit (1–2 minutes), significantly reducing documentation time .
- Interruptions in care: With ambient AI listening in, clinicians can focus on the conversation and not on typing, leading to more natural, empathetic interactions.
- Inconsistency and errors: Human-entered notes vary in detail and may omit info. AI can ensure key elements (like medication doses, allergy lists, etc.) are captured every time, and even prompt the clinician if something was left unclear.
- Administrative overload: By automating routine parts of documentation (and even things like coding and creating referral letters), these tools reduce the after-hours charting that so many providers dread.
To put it simply, the status quo of medical note-taking is unsustainable in a data-rich, fast-paced healthcare environment. AI apps for clinical notes offer a solution to streamline workflows (see more about workflow automation in our AI Toolbox category) and give clinicians some relief from the grind of paperwork.
How AI Clinical Note Platforms Help (The Solution)
AI-powered documentation tools directly tackle the above problems by serving as a digital assistant for the clinician. Here’s how they provide a solution:
- Automatic Transcription & Structuring: During a patient visit, the AI will transcribe the entire dialogue between clinician and patient using advanced speech-to-text. But it doesn’t stop there – concurrently, it analyzes the transcript with NLP to identify medical facts and organize them. For example, if a patient says “I’ve had a headache for two days” and the doctor later says “We’ll prescribe 10 mg of amlodipine,” the AI recognizes these as a symptom and a plan (medication order) respectively, and places each in the appropriate section of the note (e.g., the symptom under Subjective, the prescription under Plan). By the end of the visit, the doctor might see a nicely formatted SOAP note draft waiting in the EHR . This can save enormous time, as notes are generated in real time rather than after the fact .
- Integration with EHR systems: Leading platforms integrate directly with electronic health record software (like Epic, Cerner, etc.). This means the AI-generated notes can be synced automatically into the patient’s chart without manual copy-paste . Some solutions use APIs or middleware (for example, Nuance’s Dragon Medical One works with 200+ EHRs ). For clinicians, this integration is crucial – the AI becomes a seamless part of the workflow rather than an extra gadget. When evaluating AI tools, ensuring they connect to your existing system is key (we emphasize this in our Tips for Implementation later on).
- Real-Time Decision Support: Beyond just note-taking, some AI apps now act as a clinical aide during and after the encounter. For instance, certain platforms can summarize a patient’s past medical history and answer clinicians’ questions about the chart or medical knowledge on the fly . If a doctor asks, “AI, what were this patient’s last cholesterol levels?” an integrated assistant might fetch that info instantly. Some even provide medical reference Q&A – e.g., “What’s the recommended dosage of Drug X for pregnancy?” – drawing from trusted databases. This reduces the cognitive load on providers, who normally would click through many screens or recall guidelines from memory . In effect, the AI scribes are evolving into all-in-one clinical copilots, handling documentation plus offering decision support and information retrieval when asked.
- Consistency and Compliance: AI note platforms help standardize documentation. They can ensure each note includes necessary elements for billing and medico-legal standards (for example, including a proper review of systems, or the patient’s consent discussion, when mentioned). By using templates and consistent phrasing, the notes become more uniform and compliant with coding requirements. According to Microsoft’s Nuance, using AI yields high-quality documentation with the latest models to maintain accuracy and consistency . This consistency can improve billing outcomes and reduce claims denials since the documentation is more thorough and structured.
- Additional Outputs (Letters & Summaries): A powerful advantage is that once the AI has the encounter transcribed and understood, it can repurpose that information for other documentation needs at the click of a button. For example, Nuance’s DAX can generate referral letters or patient-friendly after-visit summaries automatically from the note . Instead of writing a separate letter to a specialist or an after-visit summary for the patient, the doctor can have the AI draft it. This multiplies the documentation value of one conversation. Imagine finishing a visit and instantly having not only the progress note ready, but also a summary to hand the patient (“Today we talked about… These are your next steps…”) and maybe even a coded bill ready for approval.
In short, these AI apps act as both secretary and analyst for the clinician. They handle the grunt work of typing and organizing notes, while also augmenting the clinical process by surfacing relevant info and ensuring nothing important gets missed. The outcome is a more efficient, accurate, and even insightful documentation process that addresses the core pain points of traditional note-taking. As a result, doctors can reclaim time and focus — which ultimately leads to better patient care (fewer distractions, more eye contact) and job satisfaction.
(Related reading: the intersection of AI and clinical practice raises interesting questions — our AI Bioethics articles discuss how tools like these balance efficiency with ethics, privacy, and the human touch in medicine.)
Key Technical Capabilities of These Platforms
Let’s delve into the tech under the hood. What makes these AI platforms capable of understanding and generating clinical notes? Several advanced technologies converge in these apps:
- Automatic Speech Recognition (ASR): The first step is turning spoken words into text. These apps use sophisticated speech-to-text engines (think along the lines of Siri or Alexa, but specialized for medical vocabulary). They can handle medical jargon, drug names, and different accents with high accuracy. Some providers rely on big cloud services (Google, Amazon, Nuance) for this; others have their own tuned models. Notably, the speech recognition is often “ambient”, meaning it works in the background without needing the speaker to pause or issue commands. For example, the platform might continuously listen during a consultation and you don’t need to say “Hey AI, start recording” – it’s just capturing naturally. Ambient ASR can also differentiate speakers (doctor vs patient) and even handle multi-party conversations (like if a family member chimes in).
- Natural Language Processing (NLP) & Understanding: Once transcribed, the raw text is analyzed by NLP algorithms. These include named entity recognition (to tag things like symptoms, medications, body parts), medical entity linking (connecting terms to medical ontologies like SNOMED or ICD codes), and context understanding (figuring out negation, certainty, timelines like “no history of diabetes” should not be tagged as a diabetes diagnosis). The AI essentially “reads” the conversation and interprets it. Modern systems use a combination of rule-based models and machine learning. Increasingly, Large Language Models play a role here: models akin to GPT-4 can maintain context over long dialogues and infer what sections of a note to populate. For instance, if a patient says “My head’s been hurting and I feel dizzy,” the AI might use an LLM to summarize that as part of HPI (history of present illness): “Patient reports two days of headache accompanied by dizziness.” The use of LLMs brings semantic comprehension — understanding the intent and nuance, not just keywords . Some custom healthcare LLMs (like Google’s Med-PaLM or others fine-tuned on medical text) are designed to be especially good at clinical language.
- Integration with EHR and Other Systems: Technically, integration is key to these platforms’ success in real clinics. Many of these AI scribes provide APIs and SDKs for EHR vendors or use standards like HL7/FHIR to push notes into the system. For example, Amazon’s HealthScribe is offered as a service for integration rather than a standalone app – it’s meant to be embedded into other clinical software . Likewise, some platforms partner directly with EHR companies (we’ve seen partnerships like Abridge with Epic, or Suki integrated into athenahealth’s mobile app). From the user perspective, integration means the AI note appears right in the patient’s chart, and the provider can sign off or edit from there. Technical capabilities here involve secure data handling (ensuring audio and text flow securely to cloud and back) and maintaining audit trails in the EHR. Many AI note apps also have voice commands to control them, often leveraging the EHR’s existing voice dictation infrastructure (for example, DAX works with Dragon Medical, which doctors may already use for voice dictation) .
- Machine Learning on Medical Data: These platforms continuously improve through training on large datasets. Companies often boast about the volume of data their AI has seen. Nuance (Microsoft) famously has a dataset of over 1 billion minutes of medical dictations and 15 million ambient conversations to train their models . This training allows the AI to get better at understanding varied medical scenarios. Machine learning models also help with things like speaker diarization (telling who said what), noise reduction (filtering out background noise in a busy ER), and even detecting non-verbal cues to some extent. For instance, an AI might flag if it “heard” a possible mention of a symptom that wasn’t followed up, just by learning from patterns in thousands of similar visits.
- Generative Text & Templates: When producing the final note, the AI uses generative techniques to compose sentences that read naturally. Early systems were template-based (inserting recognized phrases into pre-written templates, which could be a bit stiff). Now, with LLMs, many notes are generated in a more free-text style while still being structured. Generative AI allows the tool to vary phrasing, accommodate doctor-specific styles (some systems let physicians customize how formal or detailed they want notes). For example, one doctor might prefer “The patient denies chest pain or shortness of breath,” while another might want “No chest pain or SOB.” The AI can adapt formatting and phrasing accordingly, giving a level of personalization. Advanced generative capabilities also enable summarization – taking a long conversation and distilling it into a concise narrative – and concision (removing filler words, false starts in speech, etc., to produce a clean report).
- Multilingual Support: As healthcare is global, some platforms support multiple languages or bilingual conversations. For instance, DAX can handle encounters in Spanish and even allow using a translator mode . This is achieved by multilingual speech recognition and translation capabilities, ensuring that non-English interactions can still be documented accurately by the AI. This is a technical feat requiring extensive language model training across medical terms in different languages.
All these technical components work in concert inside a Clinical Notes AI app. When you use one, it feels like speaking to a recorder that magically produces a polished medical note. But under the hood, it’s a symphony of AI technologies – voice recognition turning sound to text, NLP and knowledge models understanding that text, and generative engines producing a final, structured note ready for the chart.
(For readers interested in the tech side, our Generative AI Platforms section covers how AI like GPT is being applied across industries, including healthcare.
Real-World Use Cases and Examples
How are these AI note-taking platforms actually being used in clinics today? Let’s explore a few real-world scenarios that illustrate their impact:
- Routine Documentation Automation: Use case: Primary Care Clinic. Dr. Smith, a family physician, uses an ambient AI app on her smartphone during patient visits. As she conducts a check-up, the app transcribes the entire conversation. By the end of the visit, the encounter note (history, exam findings, assessment, and plan) is already drafted. Dr. Smith spends 1 minute reviewing it, adds a quick clarification about a new referral, and signs off. Result: She saves ~10 minutes of charting per patient. Over a full day with 20 patients, that’s ~3 hours saved, which either lets her go home earlier or see a couple more patients without extending her workday. Her focus during visits is on talking and listening, not typing – something her patients notice and appreciate. In fact, in one survey 93% of patients felt their clinician was more personable and conversational when using an AI documentation assistant . The note quality is high and consistent, and Dr. Smith experiences less end-of-day fatigue.
- Telehealth and Remote Care: Use case: Telemedicine Mental Health Sessions. A mental health platform integrated Abridge (an AI notes tool) into their video call system . Therapists conducting virtual counseling sessions have the AI generate session notes automatically. Result: They saw an 80% reduction in manual note-taking effort . Therapists no longer have to type up long session narratives afterward, and can instead focus on patients during the call. Plus, the platform provides patients with a summary of the session (“In today’s therapy we discussed…”) which improves patient engagement and recall. This use case shows how AI notes are particularly helpful in talk-heavy fields like mental health, where writing detailed notes can be very time-consuming and where it’s vital to capture nuances of what was said.
- In-Patient Rounds and Summaries: Use case: Hospital Medicine. Imagine a hospitalist doing morning rounds on 10 patients with a team of trainees. Traditionally, one person scribbles down notes or they type on a workstation-on-wheels. With an AI assistant, a small microphone records the team’s discussion at each bedside. The AI transcribes the plan for each patient and generates progress notes for the day. Result: By the time rounds are done, the draft notes for all 10 patients are ready in the EHR, waiting for the physician’s review. The doctor can add any nuances and sign them. This frees up time to coordinate care with nurses or speak to patients’ families, rather than spending late mornings writing notes. Some systems also integrate with hospital workflows to pull in the latest lab results or vitals into the note automatically, giving a more comprehensive documentation without extra effort.
- Clinical Decision Support & Follow-ups: Use case: Chronic Disease Management. A diabetes specialist uses an AI platform that not only documents the visit but also analyzes the conversation for any care gaps or needed follow-ups. For example, if during the visit the patient says they haven’t had an eye exam recently and the doctor plans to refer them, the AI can pop up a reminder or even draft the referral order. Result: The doctor is less likely to forget ordering preventive measures or follow-ups discussed, because the AI “remembers” and includes them in the plan. Some AI note systems can even suggest next steps based on context (e.g., “Consider ordering lab X based on today’s findings”) . While final decisions are up to the clinician, this kind of gentle prompt can enhance decision support.
- Patient Summaries and Communication: Use case: After-Visit Summaries. By leveraging the content of the AI-generated note, clinics can provide patients with a readable summary of their visit before they walk out the door. For instance, a platform might generate a patient-friendly summary highlighting the diagnosis in simple terms, the medications prescribed, and the follow-up plan, which the doctor can hand to the patient. Result: Patients have better understanding of their care instructions (improving adherence) and feel more satisfied with the communication. According to experts, using AI to create after-visit summaries is a groundbreaking approach that can boost patient satisfaction . It ensures patients leave with answers to “What happened during my visit?” clearly documented.
These examples barely scratch the surface. We’re seeing AI notes used in specialist consultations (like oncology, where a long conversation about options can be captured verbatim), in emergency departments (to document encounters in real-time during the controlled chaos of an ER), and even in surgical settings (some surgeons dictate intra-operative findings as they go, and AI can format the op notes). The versatility of these platforms means they can adapt to many clinical scenarios, wherever documentation is needed.
One striking result across deployments is the dramatic time savings and efficiency gains. For instance, one health system study found clinicians could handle an additional patient per clinic session on average thanks to time saved on documentation . Another reported that doctors completed notes 72% faster on average with an AI assistant helping them . These are real productivity boosts, translating to either more time for patient care or more personal time for providers (both wins). No wonder many refer to ambient AI documentation as a game-changer in healthcare productivity.
Leading Market Players and Solutions
The surge in demand for AI note-taking has led to many companies and solutions entering the market. Here’s an overview of some leading platforms making waves in 2025, and what sets them apart:
Tool / Platform |
Notable Features |
Website |
---|---|---|
Nuance DAX (Dragon Ambient eXperience)Microsoft |
Ambient AI scribe integrated with EHR; uses GPT-4 (DAX Express) to generate notes automatically; can produce draft notes in seconds for clinician review . Proven at scale, with surveys showing 70% of clinicians report improved work-life balance using it . | |
Suki AI Assistant |
Voice-enabled digital assistant for doctors; offers real-time dictation to notes with commands (e.g. “Suki, create a note for physical exam”), ambient listening mode, and even handles coding (ICD-10) and voice-controlled order entry . Integrates with major EHRs (Epic, athenahealth, etc.) and claims to help complete notes 72% faster on average . | |
Abridge |
Specializes in conversation summaries for both clinicians and patients; it records visits and generates a concise clinical note for the doctor and a patient-friendly summary. Known for focusing on auditable AI (it links note text back to the original transcript for transparency) . Has partnerships to integrate into Epic and other EHRs. Great for patient communication and follow-ups. | |
Amazon AWS HealthScribe |
A cloud service (API) for developers rather than a standalone app. Provides speech-to-text and generative AI to produce transcripts and draft notes from patient-clinician conversations . Currently supports general medicine and orthopedics domains. It’s HIPAA-eligible and designed to be built into other healthcare software. Amazon’s entry signals big-tech investment here. (E.g., Amazon partnered with 3M on ambient documentation, and acquired a health startup to boost this tech .) | |
DeepScribe |
An AI medical scribe that uses ambient listening to create notes, targeted at clinics and specialties of all kinds. Emphasizes end-to-end automation with no human scribes involved (“real-time note generation with no human in the loop”). Markets itself as highly accurate and fully HIPAA-compliant. Earned a top KLAS rating for ambient AI scribes (98.8/100) – indicating strong user satisfaction. | |
Nabla Copilot |
A newer entrant using GPT-3/4-based tech (the company is based in Europe). It can listen to conversations and produce not only notes but also other outputs like prescriptions or letters automatically . Nabla’s approach is fully AI (no human scribes) and geared towards real-time note drafting with an API for easy integration into telehealth or practice software. | |
Others (Notable Mentions) |
Ambience AutoScribe – an AI scribe focused on specialty workflows, integrated in EHR with real-time notes . Augmedix – a company that historically used remote human scribes, now incorporating AI to scale (recently got major investments for ambient AI ). Eleos Health – applying AI note tech in behavioral health settings for therapy documentation. Google (Med-PaLM2) – while not a product, Google’s research with Med-PaLM LLMs is influencing next-gen clinical note capabilities with deep medical knowledge. |
(various) |
This is a rapidly growing field, so new players and features are emerging constantly. However, the ones above are widely recognized leaders or innovators as of 2025. Nuance (with Microsoft’s backing) is being adopted in large health systems; startups like Suki, Abridge, and DeepScribe are popular in clinics for their nimbleness and physician-friendly design; Amazon’s HealthScribe points to a future where any healthcare software can have note-taking AI built in via an API.
Importantly, many EHR vendors themselves are partnering with or developing AI assistants. For example, Epic (a major EHR) is collaborating with Microsoft to embed DAX directly , and athenahealth partnered with Abridge for ambient notes . This means AI clinical documentation is not a standalone niche product anymore – it’s becoming a standard feature expected in healthcare IT, much like e-prescribing or patient portals.
For a broader view of how AI is reshaping healthcare business and where investors are putting money, see our analysis in the AI Business category. Notably, over $400 million of venture funding in the last year went to startups in AI medical documentation , underlining how hot this area is.
Benefits for Healthcare Providers and Patients
Adopting AI for clinical notes offers tangible benefits for both clinicians and patients. Here are some of the key advantages observed:
Benefits for Clinicians (Doctors, Nurses, etc.)
- Significant Time Savings: Perhaps the biggest benefit – doctors reclaim hours of their day. Routine notes that used to take 10+ minutes can be completed in 1-2 minutes with AI assistance . Across a day, clinicians save an estimated 1-2 hours that would have been spent on typing and clicking . This directly translates to more time available for patient care or a better work-life balance. In one survey, 70% of clinicians said ambient AI note tools improved their work-life balance and reduced feelings of burnout .
- Reduced Administrative Burden & Burnout: By offloading the mundane documentation tasks to AI, providers experience less cognitive load. The feeling of “pajama time” (late-night charting) is less common, which improves morale. A study of Abridge’s AI noted that most clinicians felt documentation burden decreased and even reported lower burnout risk and higher job satisfaction after implementing the tool . One respondent captured it well: “AI-generated clinical notes can save physicians up to 2 hours per day — that’s time they can spend actually treating patients.” . Relief from paperwork is a huge satisfier.
- Improved Note Quality & Consistency: AI systems generate structured, comprehensive notes that follow best practices. This means important details are less likely to be missing. For instance, if the patient mentioned an allergy, the AI will make sure it’s noted. Consistent terminology and formatting reduce ambiguity. In fact, research has found a 45% improvement in documentation quality when AI tools were used . Standardized notes also help other care team members and facilitate smoother handovers between providers.
- Faster Coding and Billing: Many AI note platforms can assist with medical coding by suggesting ICD-10 codes or highlighting billable elements in the note. By structuring the note, they ensure that the documentation supports the billing codes (a common pain for clinics is missing documentation for a code). This can lead to more accurate billing and potentially improved revenue capture. Nuance reports that using AI documentation improved financial outcomes for clinics through accurate notes and even allowed 13–26% more patients seen per month per provider on average (since providers could increase throughput) . In essence, docs can see a few more patients (if they choose) without extending hours, thanks to time saved.
- Better Focus and Less Stress: During visits, clinicians using AI note-takers often report a qualitative benefit: they can focus on the patient rather than the computer. No more trying to remember everything the patient said to write it later – the AI’s got it covered. This leads to a calmer interaction. Providers feel they are truly present in the exam room or on the call. One physician noted that with an AI scribe, they felt like “the note writes itself” and they could just have a conversation. This also reduces the fear of forgetting something important that a rushed note later might miss – the AI captured it all, which the doctor can verify.
Benefits for Patients
- More Attentive, “Human” Visits: Patients notice when their doctor is engaged versus when the doctor is distracted by a screen. With AI handling the note, doctors can maintain eye contact and active listening. Patients feel heard. In a pilot with ambient AI, an impressive 93% of patients said their clinician was more personable and conversational when the technology was used . This can boost patient satisfaction scores and even clinical outcomes (patients who feel listened to are more likely to adhere to treatment plans).
- Improved Accuracy and Safety: AI-documented notes can reduce errors that might occur with hurried human documentation. For patients, that means their records are more likely to be correct and complete – allergies noted, current medications listed, symptoms accurately described. This is important for safety; e.g., if you see multiple providers, having a high-quality note from one visit helps the next provider make the right decisions. Also, AI can sometimes catch when a doctor misspoke or omitted something – for example, if the doctor forgets to address a positive finding the patient mentioned, the AI draft note might remind them of it. This “second pair of ears” can improve the quality of care.
- Clear Post-Visit Summaries and Instructions: Many AI platforms produce an easy-to-read summary for patients. Instead of cryptic doctor jargon in the after-visit summary, patients get a typed recap of what was discussed and the next steps in plain language. This helps patients recall instructions (e.g., “take these pills in the morning with food”) and understand their plan. It also benefits family caregivers who can read what happened during a visit. In surveys, patients have reacted positively to receiving these AI-generated summaries, as it increases transparency and trust (they can literally see what the doctor wrote about the visit, which used to be mostly hidden in the physician’s notes).
- Shorter Waiting Times and More Access: If doctors save time on paperwork, clinics can potentially schedule appointments more efficiently. Over weeks and months, this might mean clinics can accommodate more patients or reduce wait times for an appointment. A busy specialist could open a few extra slots, meaning patients get seen sooner for that consultation. While it’s not an immediate patient-facing feature, the increased throughput (as noted earlier, some providers managed to add 13–26 more appointments per month) can improve access to care in systems struggling with long waits . In rural or understaffed areas, this is a significant benefit.
- Continuity and Patient Engagement: Patients today also value digital engagement – some AI note systems integrate into patient portals, allowing patients to review the notes of their visit. This can empower patients to be more involved in their care, ask informed questions next time, or correct any inaccuracies they spot (which is actually a good safety check). Also, because AI can help capture holistic data (possibly from wearables, etc., in the future), patients could see a more continuous health story rather than disjointed notes. The end vision is that a patient’s health journey is well-documented and available, potentially even summarized by AI for them over time (“Your blood pressure has been stable in the last 3 visits after medication changes”).
In summary, the benefits of Clinical Notes AI apps reverberate on both sides of the stethoscope. Providers get relief and efficiency, and patients get more attentive caregivers and clearer communication. It’s a rare win-win in healthcare, a sector where innovations often help one side but burden the other (e.g., EHRs helped data gathering but added burden to doctors). That said, these benefits do depend on proper implementation and training – which leads us to the next point: not everything is perfect yet.
Read also:
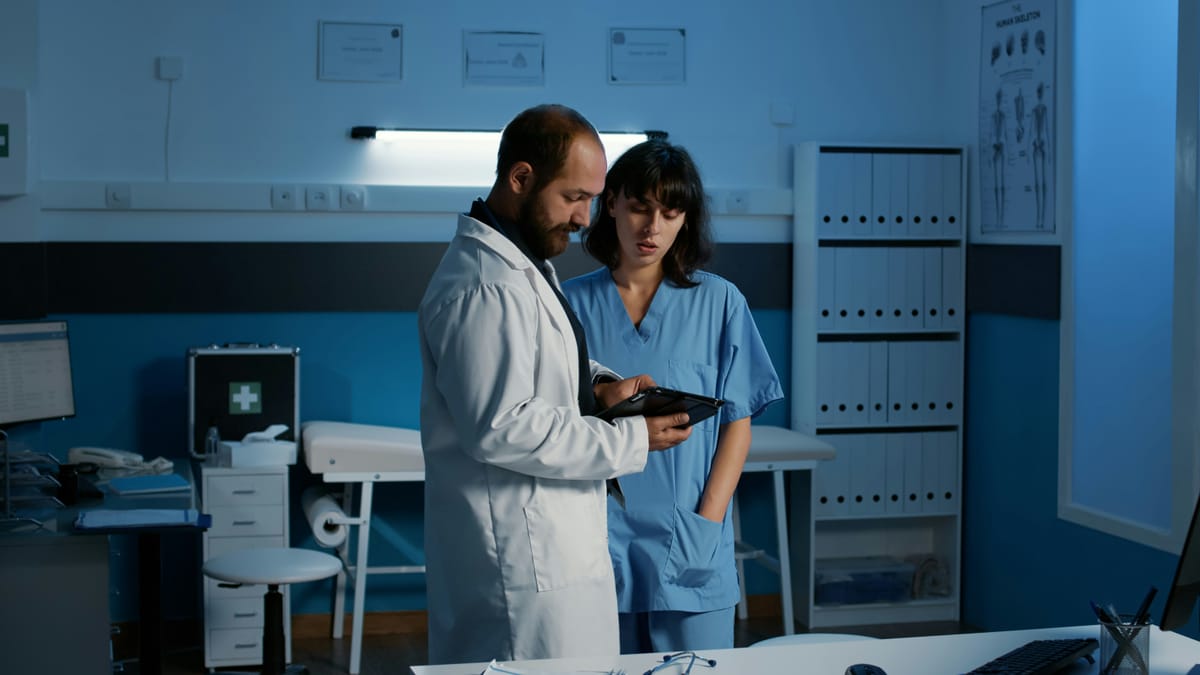
Current Challenges, Limitations, and Ethical Concerns
As promising as AI clinical documentation is, it’s important to acknowledge the challenges and limitations that come with it. Healthcare is a high-stakes domain, and introducing AI assistants must be done carefully. Here are some of the key concerns in 2025:
- Accuracy and Reliability: AI is not infallible. Speech recognition errors can occur, especially with medical homonyms or muffled speech (did the patient say “hypertension” or “hypotension”?). NLP might mislabel a piece of information – e.g., mistakenly flag a past condition as active. Therefore, human review is still required for every note. Clinicians must verify the AI-generated content. There’s a risk of over-reliance where doctors might sign off too quickly. In one study, some AI notes missed important details or had small misinterpretations, underscoring that the doctor needs to remain the final editor . If an AI consistently makes certain errors (say, confusion between drug names that sound alike), it could be dangerous if not caught. Hence, vendors are working hard on reliability, but at this stage AI doesn’t replace the clinician’s judgment – it augments it.
- Privacy and Security: Clinical conversations are among the most sensitive data. Using an AI means using recording devices, cloud services, and possibly third-party servers to process that data. Strict privacy safeguards are a must. All major platforms claim HIPAA compliance (data is encrypted, protected, not stored beyond necessary, etc.) . Amazon’s HealthScribe, for example, is described as HIPAA-eligible and emphasizes secure handling . Still, some patients (or providers) may be uneasy knowing an AI is “listening” in. Informed patient consent is a consideration – in many places, patients are informed that their visit is being recorded for documentation. Healthcare providers must ensure they and their AI vendors comply with regulations like HIPAA in the US and GDPR in Europe for data protection. There’s also the matter of data storage: will audio recordings be saved? Some services delete the raw audio once the note is generated, others might keep it for QA/training unless opted out. All these privacy details need to be transparent. (For a deeper dive into data privacy with AI, see our article on GDPR compliance in AI products – the principles apply to health data as well.)
- Bias and Fairness: AI systems can inadvertently carry biases from their training data. If the data used to train the note generation models under-represents certain dialects or patient groups, the AI might perform worse for those populations. For example, an AI might more frequently misunderstand a heavy accent or fail to capture culturally specific ways of describing symptoms. Also, there’s concern that if an AI note system is trained on past documentation, any biases in what doctors tend to note (or not note) could be perpetuated. For instance, studies show certain populations have less detailed notes due to various systemic issues; an AI learning from those could consider that normal. Addressing bias requires continuous evaluation and feeding diverse data for training. Some vendors incorporate feedback loops to improve performance across demographics. Still, it’s an evolving challenge: AI is only as fair as the data and design behind it. Healthcare providers should ask vendors about how they mitigate bias.
- Overdependence and Deskilling: There’s a subtle risk that clinicians might lose some documentation skills or awareness if they rely too much on the AI. For example, writing a note forces a doctor to think through the case and ensure all points are covered – if the AI does it, a more passive review might miss something. Or younger doctors might not develop note-writing proficiency (though one could argue that’s not a bad thing if AI does it). Additionally, some fear that if clinicians become dependent on AI to recall everything, their own memory or attentiveness might wane (“the AI will catch it, so I won’t write it down myself”). It’s important for training programs and hospital policies to emphasize that the clinician is still responsible for the content. AI should be a tool, not a crutch. Maintaining a healthy skepticism and double-checking the AI output is part of the new skill set.
- Workflow Integration and Adoption Hurdles: Implementing AI note systems isn’t just plug-and-play. It requires integration into existing IT systems and workflows. Some clinics may face technical challenges or costs in doing so. There can be resistance from staff, especially those less tech-savvy or who have had bad experiences with earlier voice recognition tech. Training is needed to get comfortable with correcting AI notes via voice or learning new software interfaces. There’s also the issue of infrastructure: an always-listening AI might need good quality microphones in exam rooms, robust internet connectivity (especially for cloud-based services), etc. In rural or resource-limited settings, these prerequisites can be a barrier . The adoption curve can be steep for some – change management and demonstrating the value are crucial to get everyone on board.
- Cost and ROI: These advanced AI platforms are not cheap. Many operate on subscription models that can cost hundreds of dollars per month per provider (for example, some charge ~$150–$300 per month per clinician seat; enterprise deals vary). For smaller practices, the cost might seem high. The promise is that the time saved (or extra billing captured) offsets this. In fact, Suki claims using their AI can deliver a 9x ROI in the first year by accounting for time saved and increased revenue from more patients or services . Each practice will have to evaluate if the financial trade-off makes sense. Over time, competition and scale might bring prices down, but currently the best solutions are a serious investment.
- Legal and Ethical Considerations: Who is responsible if the AI makes an error in the note that leads to a patient harm? Legally, the clinician signing the note is responsible, but what if an AI introduces something the clinician didn’t actually say and they overlook it? These grey areas are being discussed in medical ethics circles. There’s also the question of patient consent we mentioned: ethically, patients should be aware if their data is being processed by AI, even if it’s behind the scenes. Most places treat the AI like a technical aide, not much different from the EHR itself, but transparency is key to trust. From an ethical standpoint, clinicians must also be wary of over-trusting the AI’s suggestions (e.g., if an AI suggests a follow-up test, the doctor should still use clinical judgment to decide if it’s appropriate).
Despite these challenges, the trajectory seems to indicate that the benefits outweigh the drawbacks as the technology matures. It’s telling that many experts view AI note-taking not as a passing trend but as an inevitable transition in medicine . Each limitation is actively being worked on: accuracy improves with better models and more data, privacy with strong encryption and policies, bias with conscious engineering, etc. We are still in early days, and healthcare is rightly cautious. The approach being taken is often to start with a pilot program (test the AI with a few users, measure impact, adjust) rather than a big bang deployment. As best practices form, we’ll likely overcome many of these issues.
(Ethical side note: For those interested in how AI is handled responsibly, especially in fields like healthcare, our AI Bioethics tag covers the debates on privacy, consent, and the human-AI balance in detail.)
Future Outlook: AI’s Growing Role in Clinical Documentation
Looking ahead, the future of Clinical Notes AI is incredibly exciting. The year 2025 already finds us at a point where AI can draft a decent clinical note; in the next few years, we’ll see these tools become even more powerful, more intuitive, and more ubiquitous. Here are some trends and innovations on the horizon:
- Deeper Integration and “Invisible” Documentation: The ultimate vision is that documentation becomes a byproduct of care, not a separate task. We’re moving toward a continuous clinical record. Future systems might automatically pull in data from wearables, patient apps, or other sources along with the visit conversation, and compile a comprehensive note or health timeline . The AI could, say, integrate your smartwatch’s BP readings into your progress note if relevant. In-person visits, telehealth calls, remote monitoring – all could feed into an AI that maintains your health story in real time. For doctors, documentation might shift to a supervisory role: they ensure the AI-curated record is accurate, rather than writing everything themselves. This could make the medical record far more rich and useful, providing insights at a glance (as one can imagine the AI summarizing “here’s how the patient’s asthma has trended over the last year across all encounters”).
- Advanced LLMs and Clinical Reasoning: Large Language Models are getting more specialized for healthcare. We will likely see versions of GPT or similar that are fine-tuned not just to write notes, but to perform some level of clinical reasoning on the fly. For instance, an AI might automatically generate a differential diagnosis list in the note (e.g., if a patient’s symptoms are not clear-cut, the AI might list possible causes as part of the Assessment). Or it could draft the Assessment/Plan in more detail by synthesizing guideline information. Google’s Med-PaLM 2 has shown ability to answer medical questions at a level approaching clinicians in some cases – such capabilities might be embedded to ensure the note includes recommended care steps or checks against best practices. Essentially, documentation AI could evolve from passive scribe to active assistant that can suggest diagnoses or alert if something in the conversation sounds concerning that wasn’t addressed (almost like an AI co-pilot double-checking the clinician’s decisions). This crosses into decision support territory heavily, and will have to be balanced (doctors won’t want an overeager AI interrupting with suggestions constantly). But done right, it could improve care quality.
- Multimodal Documentation: So far we talked about text, but future clinical note AIs might incorporate images or other data into the documentation workflow. For example, if during an exam a dermatologist takes a photo of a skin lesion, the AI could automatically embed that image in the note and even generate a description (“Image of 5mm mole on left forearm attached”). Or in radiology, an AI might take dictations and link to the actual scans. Multimodal AI (which can handle text, images, and more) will allow documentation to go beyond text, perhaps generating patient graphs, flowcharts of treatment progress, etc., as part of the record.
- Personalization and Learning from the Clinician: Future systems will likely adapt to individual clinicians’ styles even more. Much like how your smartphone adapts to your voice or typing quirks, an AI note assistant could learn a doctor’s preferred phrasing, how verbose or concise they like their notes, and adjust accordingly without needing explicit instructions each time. We might also see AI analyzing a clinician’s past notes to create a profile — e.g., Dr. Jones always writes very detailed plans, so the AI will try to do the same; Dr. Lee is brief, so the AI keeps it short unless more detail is needed for clarity.
- Greater Patient Interaction with Notes: As notes become more accurate and instantly available, patients might get more direct access. Some foresee a time when patients have their own AI medical assistant that can explain the doctor’s note to them, or even liaise with the doctor’s AI (with permission, of course). For instance, a patient’s digital health app could summarize the latest note in lay terms and let the patient ask follow-up questions (“What did the doctor mean by benign prostatic hyperplasia?” – and it can answer from reputable sources). This triad of patient, clinician, and AI assistants collaborating might improve understanding and continuity dramatically.
- Regulatory and Standardization Developments: We’ll likely see professional and regulatory bodies provide guidelines on AI documentation. For example, standards on how AI involvement in notes should be documented (some EHRs now tag notes that were AI-generated or have a log of edits, etc.). Medical schools and training programs may also formally include training on using AI tools effectively and responsibly. On the legal side, as these tools prove themselves, some documentation requirements might be relaxed (e.g., if AI ensures completeness, maybe visit note templates become simpler). There’s talk of eventually achieving real-time coding such that doctors don’t even think about billing – the AI handles it and regulators accept that because it’s proven accurate.
- Wider Adoption and Democratization: If 2023–2025 was about early adopters and pilot programs, the rest of the decade will see mainstream adoption. By 2030, it may be unusual not to have some form of AI assisting with notes in healthcare settings. The market prediction of $187 billion for AI in healthcare by 2030, with a big chunk in documentation, suggests huge growth . Competition will probably drive costs down and make solutions accessible even to small clinics or practices in developing regions (perhaps through smartphone-based systems). Open-source models might also emerge, allowing institutions to run AI note-taking on their own private servers, addressing some data privacy concerns.
Ultimately, the future outlook is that AI will make clinical documentation ambient, automated, and almost invisible. Doctors might come to view writing notes the same way we view using paper charts today – outdated and inefficient. The relationship between doctor and patient could shift to more direct interaction because the “paperwork barrier” is lifted by technology. As one forward-looking perspective put it: in the future, “Less typing, more talking. Less stress, more accuracy. Less paper, more insight.” . The hope is that AI frees healthcare professionals to be the healers and thinkers they trained to be, rather than data clerks, while still capturing all the necessary information to deliver top-notch care.
Of course, reaching that ideal will require careful navigation of the challenges we discussed. But given the rapid advancements and the positive early experiences, the trend is clear: AI is here to stay in clinical documentation, and it’s only getting smarter.
Frequently Asked Questions (FAQ)
What are AI clinical note apps in simple terms?
They are AI-powered assistants that listen to doctor-patient visits and automatically write the medical notes. Instead of the doctor typing everything, the app transcribes the conversation and produces a formatted record of the visit. It’s like having a smart scribe in the room who knows medical terminology and can draft your notes for you.
How accurate are AI-generated clinical notes?
In general, quite accurate, but not perfect. The best systems achieve highly accurate transcriptions and can correctly identify medical details most of the time. However, errors or omissions can occur, so clinicians must review and edit the AI’s output . Accuracy keeps improving as the AI learns from more data. In practice, doctors find they only need to make minor tweaks in many cases, but they cannot rely 100% on the AI without oversight yet.
Are these AI note-taking tools secure and HIPAA-compliant?
Yes – leading platforms place heavy emphasis on privacy and security. Data is usually encrypted in transit and storage, and reputable services are HIPAA-compliant, meaning they meet healthcare privacy regulations . For example, Amazon HealthScribe is described as HIPAA-eligible and keeps data secure . Hospitals and clinics also sign agreements with vendors to ensure patient data is protected. Patients are often informed that an AI may be used in their care for transparency. Always choose solutions that explicitly state their compliance (HIPAA, GDPR, etc.) if privacy is a concern.
Will AI note apps replace medical scribes or doctors?
They are likely to replace the role of human scribes in many settings, yes. In fact, many clinics that used to hire scribes are transitioning to AI for note-taking because it’s faster and more cost-effective . However, they will not replace doctors – the AI doesn’t make medical decisions or interact with patients on the human level. It’s a tool that assists doctors. Doctors will still be needed to provide care, use judgment, and verify the AI’s work. The AI might handle the grunt work of documentation better than a person, but the clinician remains in charge of patient care.
Can these AI platforms integrate with any EHR system?
Most major AI clinical note solutions offer integration with popular EHRs like Epic, Cerner, Allscripts, athenahealth, etc. Some, like Nuance’s, integrate via Dragon Medical which already hooks into EHRs . Others have partnerships or use standards (FHIR APIs) to plug notes into the EHR. If an EHR is older or more niche, integration might require custom work. Always check if the AI vendor supports your specific EHR. In many cases, the AI can at least output a note that can be pasted or imported if direct interface isn’t available. The trend is improving integration as EHR companies themselves are welcoming these tools.
How do patients feel about being recorded by an AI?
Patient acceptance has been generally positive, especially when it’s explained that the AI helps the doctor focus on them. Many patients appreciate their doctor not turning away to type. In some pilots, patients reported a better experience, saying the doctor seemed more attentive and “present” . There is still a need to ensure patients are comfortable – usually, the doctor will mention something like “This device is securely recording so I can have our conversation documented. Let me know if you have concerns.” With that, most patients agree. Especially when they get a benefit (like a summary of the visit), patients tend to see the AI as a helpful part of the clinic team.
How can a clinic start using AI for notes?
The best approach is to start with a pilot program. Identify a trustworthy AI notes platform, ensure it’s compatible with your systems, and have a few willing clinicians try it out with training. Evaluate the impact on their documentation time and note quality. Provide feedback to the vendor and tweak any settings. Gradually roll it out to more providers if the pilot goes well. Key steps include securing IT support for integration, training staff on how to use and edit AI-generated notes, and informing patients. Many vendors offer trial periods or demos. It’s wise to measure things like time saved per note or provider satisfaction before and after – these help build the case for broader adoption.
We hope this comprehensive overview answered your questions about what Clinical Notes AI apps are, how they work, what features to look for, and which solutions are leading the way in 2025. AI-driven note-taking is poised to shift medical documentation from a tedious chore to an automated assist, allowing clinicians to spend more time with patients and less on screens. As you consider adopting these tools, remember to pilot thoughtfully, keep an eye on data security, and involve your team in the transition. The healthcare industry is embracing these innovations quickly – staying informed (and reading articles like this!) will help you ride the wave of change effectively. With the right approach, AI clinical notes can be a win for productivity, professional satisfaction, and patient care quality.
Ready to reclaim your time and enhance your practice with AI? It might be the perfect moment to explore a clinical documentation assistant in your workflow. Early adopters have reported life-changing reductions in after-hours charting and a rekindled focus on the patient-doctor relationship. The days of drowning in paperwork could finally be ending.
For further insights into healthcare AI and other productivity-boosting tools, feel free to explore our other in-depth articles and resources. And if you found this guide useful, consider signing up for HumAI.blog to get weekly insights on how AI is transforming work and life in various fields, including healthcare. Together, we’ll keep navigating the exciting intersection of technology and human care.