In the rapidly evolving landscape of artificial intelligence, hardware has become the critical foundation enabling the AI revolution. As we navigate through 2025, AI hardware has transcended from specialized components for tech enthusiasts to essential tools transforming industries, homes, and personal devices worldwide. This comprehensive guide explores the latest AI hardware trends, breakthrough gadgets, and innovations that are reshaping how we interact with technology.
Whether you're a tech professional seeking the most powerful edge AI development platforms, a consumer interested in the latest AI-enhanced gadgets, or a business leader evaluating hardware investments, this guide provides the insights you need to navigate the complex world of AI hardware in 2025.
What Is AI Hardware and Why It Matters in 2025
AI hardware refers to the specialized physical components designed to efficiently run artificial intelligence workloads. Unlike general-purpose computing hardware, AI-specific hardware is optimized for the unique computational demands of machine learning algorithms, neural networks, and other AI applications.
The evolution of AI hardware has been dramatic, shifting from centralized cloud processing to distributed edge computing. This transformation has been driven by several factors:
- Privacy concerns: Processing data locally reduces the need to send sensitive information to the cloud
- Latency requirements: Real-time applications demand immediate processing without network delays
- Connectivity limitations: Edge AI enables operation in environments with limited or no internet access
- Energy efficiency: Specialized hardware reduces power consumption for AI workloads
In 2025, AI hardware has become a strategic differentiator across industries. According to Deloitte's tech trends report, "Hardware is eating the world," with enterprise infrastructure once again becoming a critical competitive advantage after years of being relegated to utility status. The global data center capacity is expected to double by 2027, largely driven by AI demand.
For consumers, businesses, and developers alike, understanding the AI hardware landscape is no longer optional—it's essential for making informed technology decisions.
Top AI Hardware Trends Shaping 2025
Custom AI Chips and Specialized Silicon
The surge in custom AI chips represents one of the most significant hardware trends of 2025. As AI models have grown increasingly complex, general-purpose processors like CPUs and GPUs have reached their limits. This has spurred the development of specialized silicon designed specifically for AI workloads.
Neural Processing Units (NPUs), Tensor Processing Units (TPUs), and AI accelerators are now common components in everything from smartphones to data centers. These custom chips deliver faster processing, lower latency, and reduced energy consumption compared to general-purpose processors.
NVIDIA remains the dominant player in the AI chip market with its H100 Tensor Core GPU and Blackwell architecture, but competition is intensifying. AMD is gaining ground with its MI300 series, offering impressive price-to-performance ratios. Meanwhile, smaller companies like Cerebras, Graphcore, and SambaNova are making headlines with innovative approaches to AI acceleration.
Large tech companies are increasingly developing their own custom silicon to reduce dependence on third-party vendors. Google's TPU v5p and AWS Trainium are notable examples of this trend.
Edge AI vs. Cloud Processing
The balance between edge and cloud AI processing continues to evolve in 2025. Edge AI—processing data directly on devices without requiring internet connectivity—offers advantages in speed, security, and privacy. Meanwhile, cloud AI provides greater computational resources for complex models.
Hyperscalers like AWS, Azure, and Google Cloud are doubling down on AI-optimized cloud infrastructure, offering end-to-end platforms that include specialized hardware, developer tools, and pre-trained models. These comprehensive ecosystems are attracting enterprises looking for streamlined AI deployment options.
Simultaneously, "local AI" is booming, particularly in smartphones, wearables, and autonomous vehicles. Apple's A18 Bionic, Google's Tensor G4, and Qualcomm's Snapdragon X80 all integrate specialized NPUs that support on-device AI processing, eliminating the need for constant cloud connectivity.
The most effective AI implementations in 2025 often employ a hybrid approach, balancing edge and cloud processing based on specific use case requirements.
Neuromorphic and Quantum Computing Advances
While still emerging technologies, neuromorphic and quantum computing are beginning to influence the AI hardware landscape in 2025.
Neuromorphic chips, designed to mimic the brain's neural structure, excel at pattern recognition and sensory processing tasks while consuming minimal power. Intel's Loihi neuromorphic processor is finding applications in edge AI scenarios where ultra-low power consumption is critical, such as in vehicles, smart sensors, and wearables.
Quantum computing remains primarily in the research phase but is showing promise for specific AI optimization tasks, particularly in reinforcement learning and cryptographic analysis. Companies like IBM are developing hybrid AI-quantum systems that could eventually solve problems beyond the capabilities of classical computing.
Energy Efficiency and Sustainability
The environmental impact of AI hardware has become a major concern in 2025. As data centers expand to meet AI demand, their energy consumption is pushing power grids to their limits globally.
According to IEEE Spectrum's AI Index 2025, the carbon emissions from training frontier AI models have steadily increased over time. Meta's Llama 3.1 resulted in an estimated 8,930 tonnes of CO2 emitted—equivalent to the annual carbon footprint of approximately 496 Americans.
This environmental challenge has spurred innovations in energy-efficient AI hardware and cooling technologies. NVIDIA's latest chips offer significantly improved energy efficiency compared to previous generations, and data centers are increasingly adopting liquid cooling, advanced power management systems, and AI-optimized rack designs to reduce their carbon footprint.
The push for sustainable AI has also led many companies to explore alternative energy sources, with nuclear power emerging as a popular option for providing reliable, carbon-free electricity to power AI infrastructure.
Best Consumer AI Gadgets of 2025
Halliday AI Glasses with Invisible Display
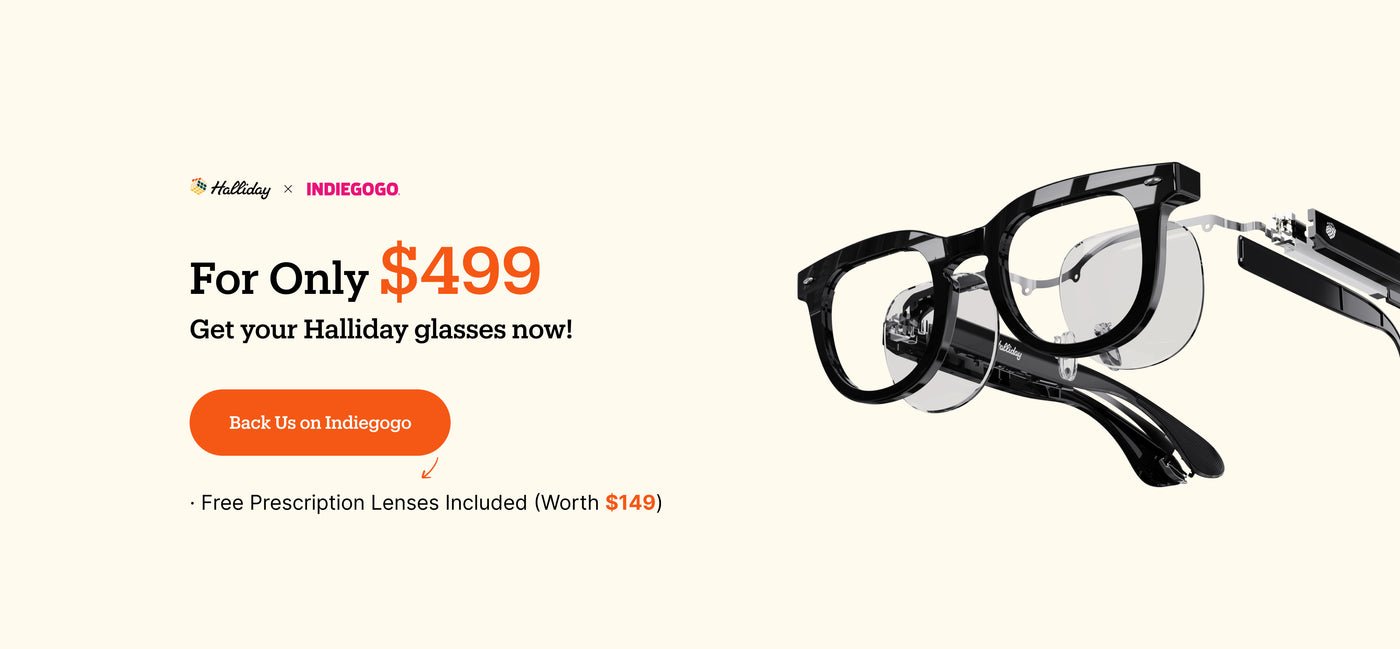
Halliday's AI Glasses represent a breakthrough in wearable AI technology, featuring what the company calls an "invisible display." Unlike traditional smart glasses that incorporate displays into the lenses, Halliday's approach integrates the world's smallest optical module (just 3.6mm) into the frame itself.
The result is a pair of glasses that look like ordinary eyewear but provide a field of view similar to a 3.5-inch screen visible only to the wearer. At just 35 grams, they're noticeably lighter than competitors like Meta's Ray-Ban smart glasses.
Key features include:
- Real-time translations in over 40 languages
- Teleprompter functionality
- Note-taking capabilities
- Notification display (texts, music, etc.)
- Turn-by-turn navigation
- 12-hour battery life
- Prescription lens compatibility
The Halliday Glasses retail for $499, with early adopters able to secure them for $369 through a reservation system. Their combination of discreet design, practical functionality, and all-day wearability makes them one of the most impressive consumer AI hardware products of 2025.
Bee AI Wearable
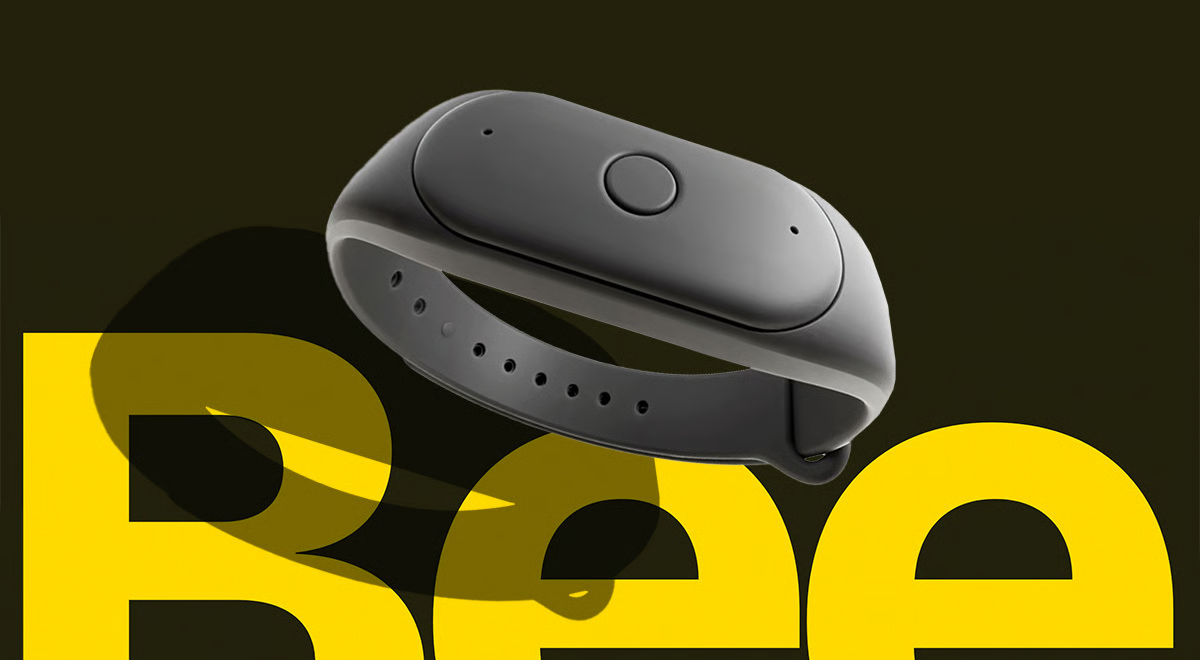
The Bee AI wearable represents a unique approach to personal AI assistance. This wristband continuously listens to your conversations throughout the day (unless manually paused) to build a comprehensive understanding of your life and interactions.
The device provides AI summaries of conversations, transcripts, and actionable insights based on what it hears. Users can chat with the built-in AI assistant to learn more about their day, reference specific conversations, or receive suggestions for behavioral improvements.
With a seven-day battery life and integration with services like Google Calendar and Gmail, the Bee AI wearable offers a novel approach to personal memory augmentation and productivity enhancement. The company emphasizes privacy protections, stating that users control their memories, no humans can access the data, the information won't be sold or trained on, and audio isn't saved.
The Bee AI wearable is available for $50, making it one of the more affordable entry points into wearable AI technology.
JBL Tour One M3 AI Headphones
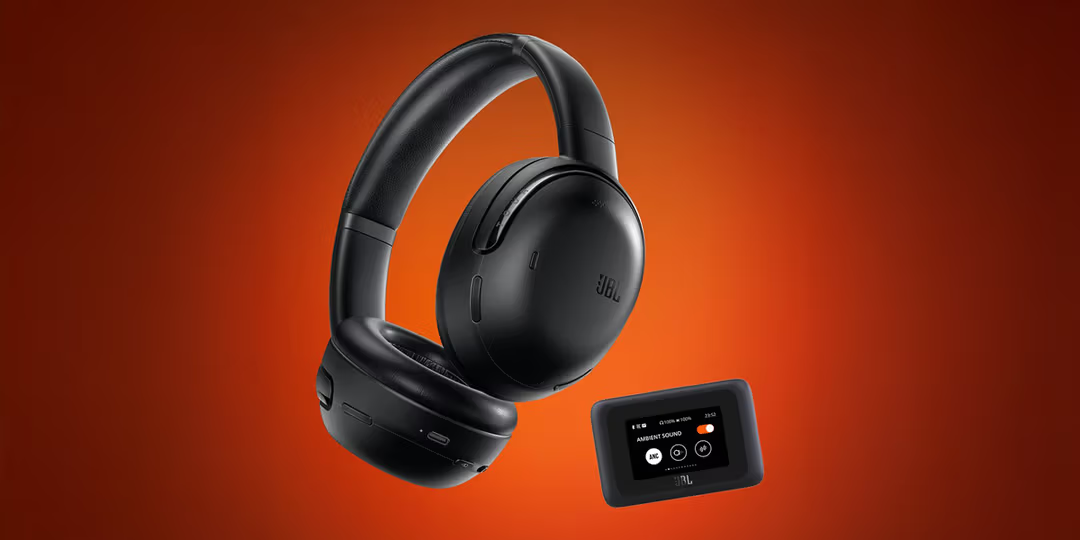
JBL's flagship Tour One M3 headphones represent the cutting edge of AI-enhanced audio technology in 2025. These premium headphones push the boundaries of connectivity by adding a touchscreen Bluetooth transmitter similar to the one in JBL's Tour Pro 3 earbuds.
This innovation allows users to connect to in-flight entertainment systems, achieve lossless audio through both 3.5mm and USB-C wired connections, and manage their listening experience with unprecedented control.
The headphones feature advanced AI algorithms that optimize call quality, automatically adjusting noise cancellation and voice pickup based on environmental conditions. The result is crystal-clear communication even in challenging acoustic environments.
With adaptive sound optimization that continuously analyzes and adjusts audio based on the user's hearing profile, ear shape, and listening preferences, the Tour One M3 delivers a personalized audio experience that evolves over time.
Shokz OpenMeet AI Headset
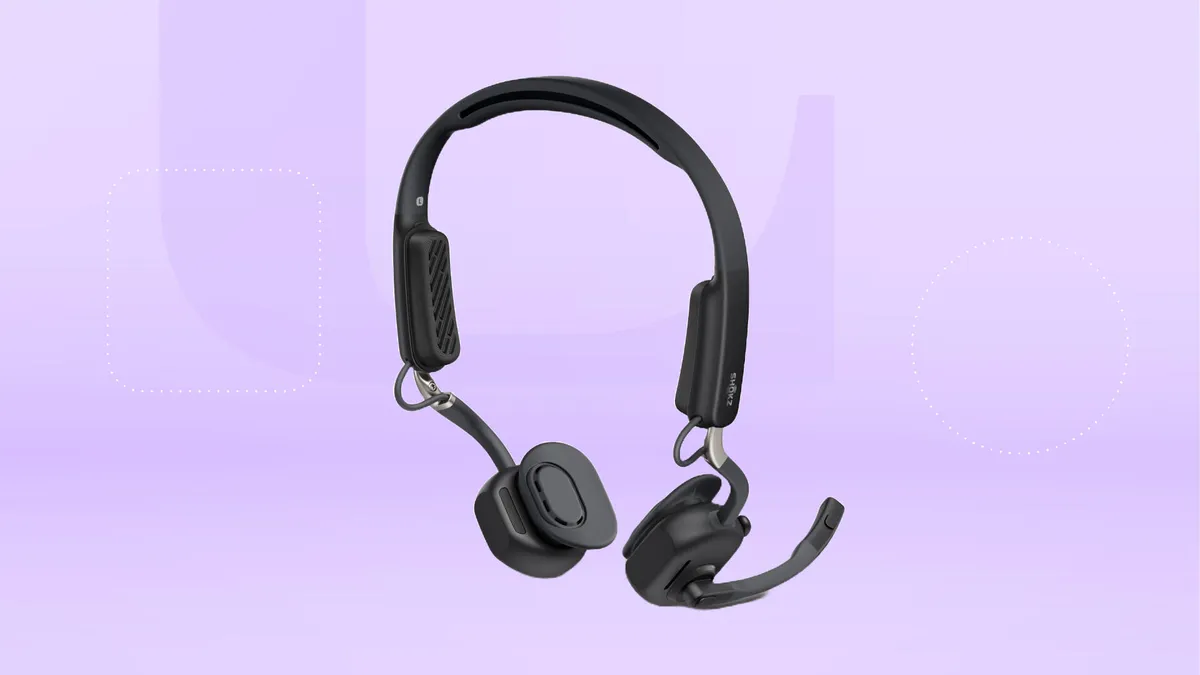
Shokz has leveraged their bone-conduction technology to create the OpenMeet, an AI-powered headset designed specifically for remote work environments. The featherlight, comfortable design allows for all-day wear, even with glasses.
The standout feature is the noise-cancelling boom microphone enhanced by AI algorithms that effectively filter out background noise in busy workplaces or cafés. The open-ear design ensures users remain aware of their surroundings while enjoying clear audio and call quality.
The headset's AI assistant can transcribe conversations in real-time, schedule follow-ups based on meeting content, and provide summaries of key discussion points. For remote workers who need to stay connected without isolating themselves from their environment, the Shokz OpenMeet represents an ideal balance of functionality and comfort.
Leading Edge AI Development Hardware
NVIDIA Jetson AGX Orin
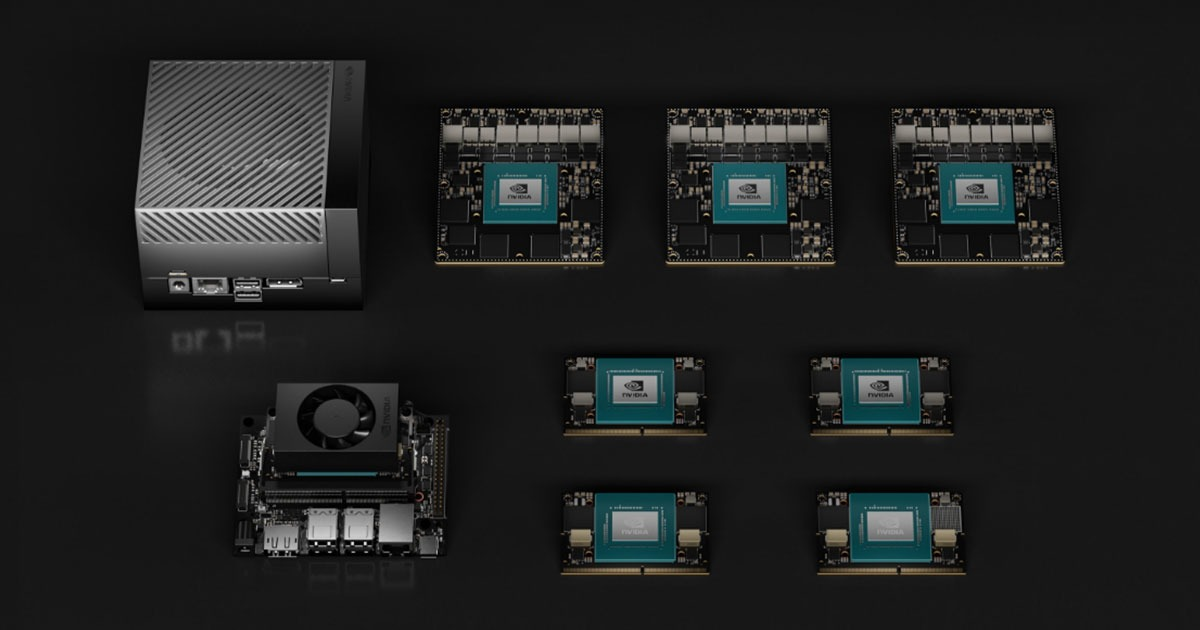
The NVIDIA Jetson AGX Orin stands as the flagship edge AI computer for developers and manufacturers in 2025. Delivering up to 275 trillion operations per second (TOPS), it offers 8 times the performance of the previous generation for multimodal AI inference.
This high-end edge AI module features:
- 12-core Arm Cortex-A78AE CPU
- 2048-core NVIDIA Ampere GPU with 64 Tensor Cores
- Up to 64GB of LPDDR5 memory
- Configurable power consumption from 15W to 60W
The Jetson AGX Orin is designed for demanding edge applications such as autonomous robots, drones, and advanced computer vision systems. Its comprehensive software stack includes pre-trained AI models and reference workflows that accelerate development for generative AI and other edge applications.
For companies developing next-generation autonomous systems, the Jetson AGX Orin provides server-class AI performance in a compact form factor suitable for deployment in the field.
Google Coral Dev Board
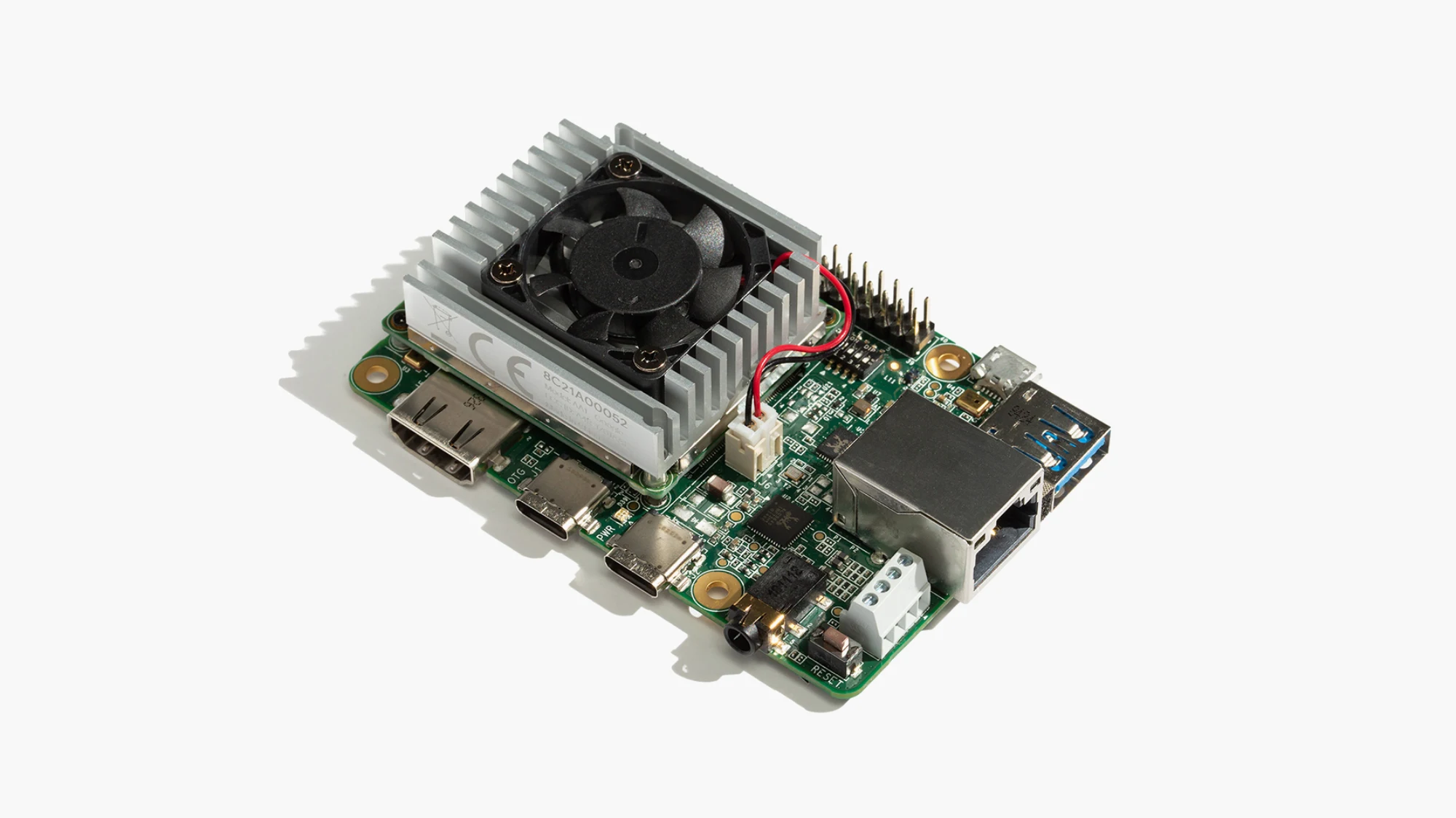
Google's Coral Dev Board features the company's custom Edge TPU (Tensor Processing Unit) ASIC as a co-processor specifically designed for on-device machine learning inference. The Edge TPU delivers 4 TOPS of int8 performance at just 2 watts, resulting in an impressive efficiency of 2 TOPS per watt.
This efficiency enables the Coral to execute state-of-the-art vision models like MobileNet V2 at nearly 400 frames per second in real-time. The board's system-on-module includes:
- NXP i.MX 8M SoC (quad Cortex-A53 CPU)
- 1GB or 4GB RAM options
- Full Linux OS (Mendel Debian)
- Starting price of $129.99
The Coral Dev Board is optimized for AI at the edge in small form factors and power-constrained environments. It's particularly popular for vision-based IoT applications such as smart security cameras, image recognition sensors, and portable ML devices.
Developers appreciate its ease of use with TensorFlow Lite models and Python APIs, making it an excellent choice for prototyping edge AI solutions before scaling to production.
Qualcomm Robotics RB5 Platform
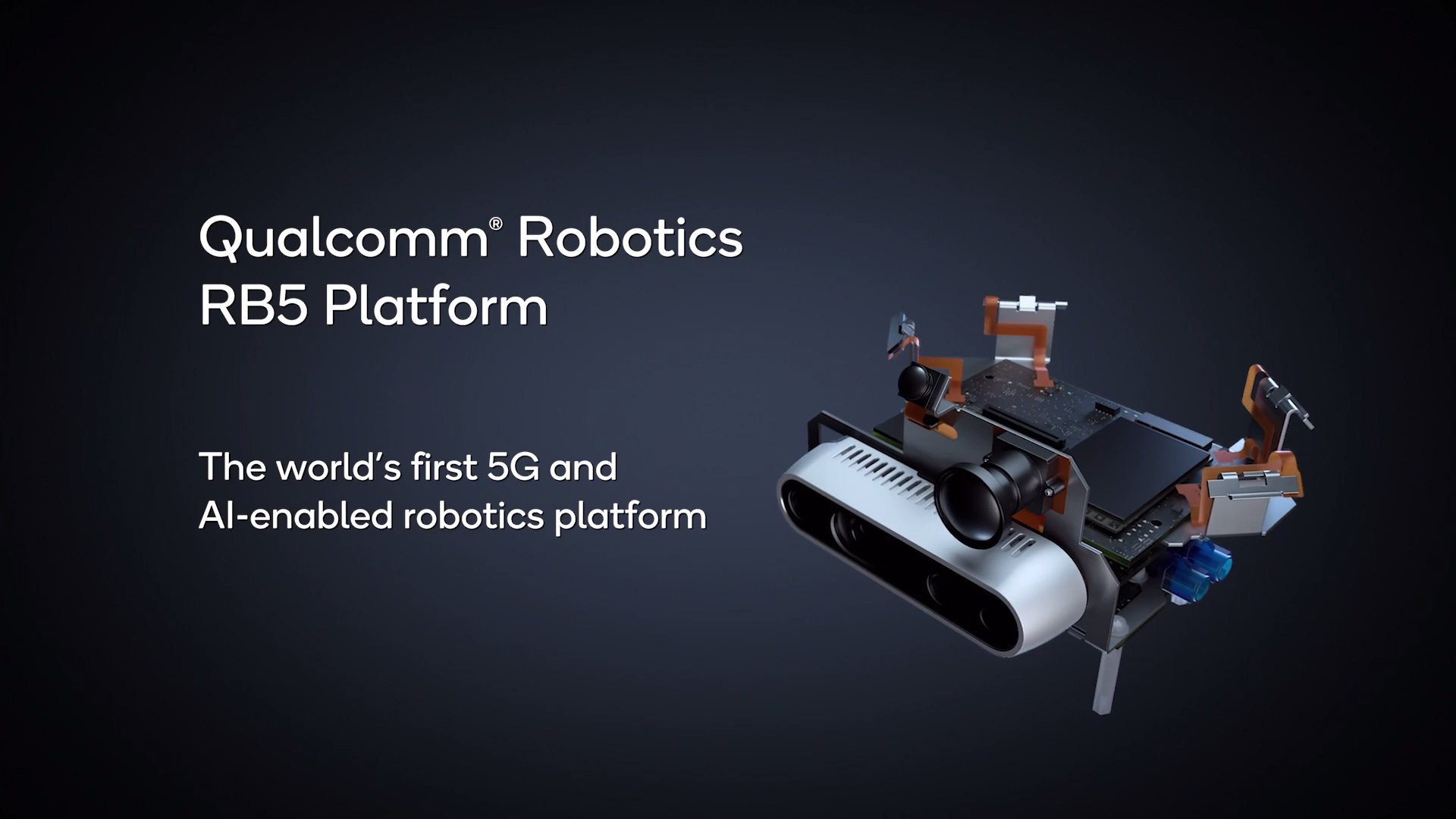
Qualcomm's Robotics RB5 Platform combines high-performance AI computing with advanced connectivity options, making it ideal for next-generation robots and autonomous systems. At its core is the Qualcomm QRB5165 SoC, featuring:
- Octa-core Kryo 585 CPU
- Adreno 650 GPU
- 5th-generation Qualcomm AI Engine with Hexagon Tensor Accelerator
- 15 TOPS of on-device AI performance
- Support for up to 7 concurrent cameras
- Optional 5G connectivity
The platform's powerful heterogeneous computing capabilities (CPU, GPU, DSPs, ISP) and dedicated AI cores make it perfect for mobile robots performing sensor fusion, real-time object detection, SLAM (simultaneous localization and mapping), and path planning directly on the device.
In industrial settings, RB5-based robots can use computer vision for quality control while maintaining secure local processing of sensitive manufacturing data. The platform's 5G capabilities also enable low-latency remote operation and cloud coordination when needed.
AMD Xilinx Kria K26 SOM
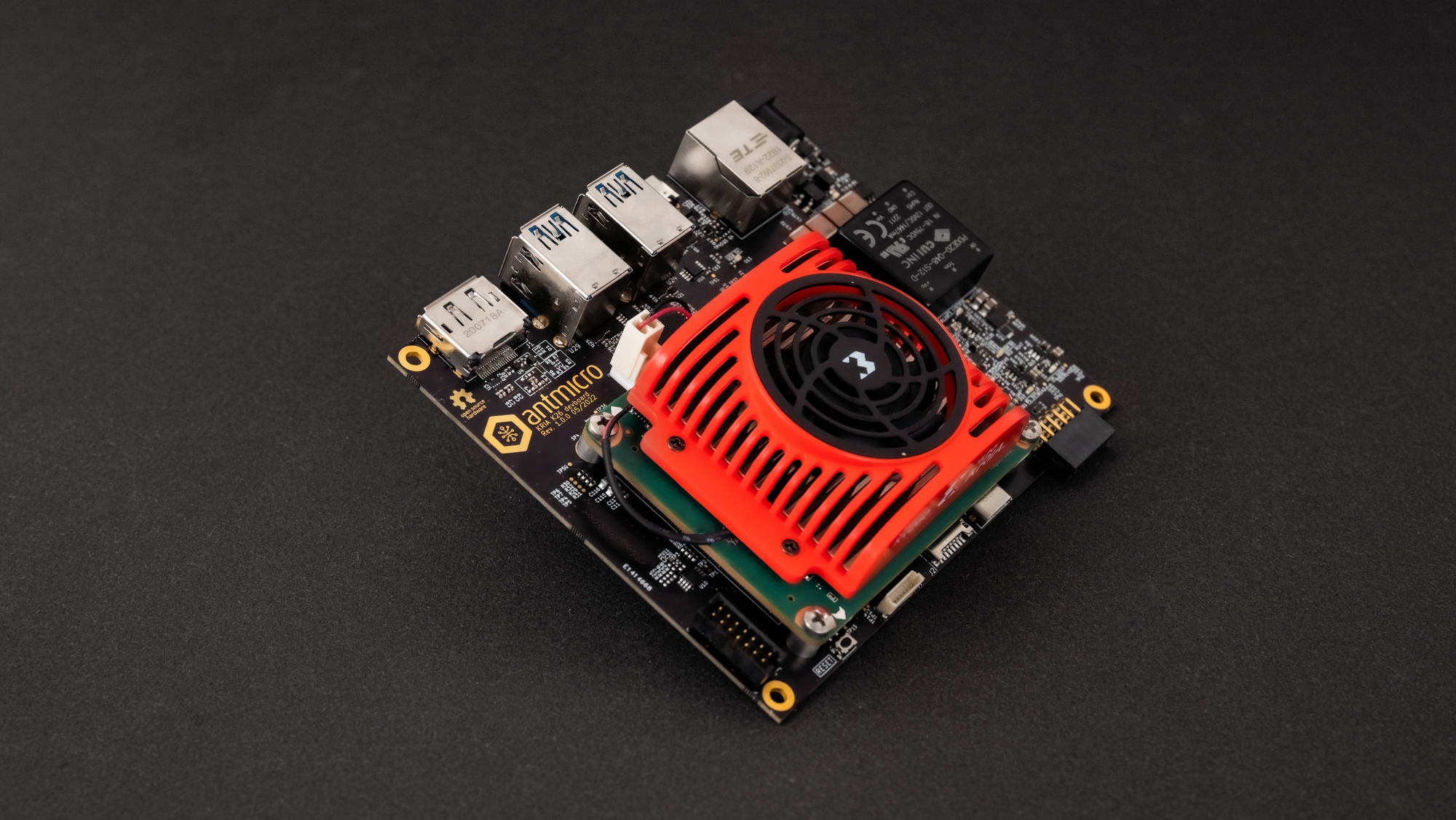
The Kria K26 system-on-module from AMD Xilinx targets vision AI and robotics applications with a unique combination of processing power and adaptability. Built around a Xilinx Zynq UltraScale+ MPSoC, it combines:
- Quad-core Arm Cortex-A53 CPU
- Programmable FPGA logic and AI engines
- Deep learning processor (DPU) delivering 1.4 TOPS
- Dual-core real-time Arm R5F processor
- H.264/H.265 video codec
The FPGA fabric allows developers to implement custom image processing pipelines or sensor interfaces alongside AI inference, making the Kria K26 highly adaptable to specific vision or robotics tasks. The KV260 Vision AI Starter Kit pairs the K26 SOM with a carrier board that supports up to 15 cameras via MIPI CSI and 4K display output.
AMD provides pre-built accelerated applications and ROS 2 support to speed up prototyping, making the Kria K26 an excellent choice for computer vision applications in industrial and smart city contexts.
Real-World Applications and Use Cases
Smart Cities and Infrastructure
AI hardware is transforming urban environments in 2025, with edge devices processing data from thousands of sensors to optimize traffic flow, reduce energy consumption, and enhance public safety.
Smart traffic management systems powered by edge AI cameras can detect congestion patterns and automatically adjust signal timing to improve traffic flow. These systems operate locally, ensuring functionality even during network outages and protecting citizen privacy by processing data on-device.
In public transportation, AI-enhanced predictive maintenance systems use vibration sensors and edge processors to detect potential equipment failures before they cause disruptions. The combination of real-time processing and machine learning enables more reliable service while reducing maintenance costs.
Urban infrastructure monitoring has also been revolutionized by AI hardware. Drones equipped with edge AI processors can inspect bridges, buildings, and other structures, identifying potential issues that might be missed by human inspectors. The ability to process high-resolution imagery directly on the drone eliminates the need to transmit massive data sets to central servers.
Healthcare and Medical Applications
The healthcare industry has embraced AI hardware to improve diagnostics, patient monitoring, and treatment planning. Edge AI devices are particularly valuable in healthcare settings where privacy concerns and the need for real-time analysis are paramount.
AI-powered diagnostic tools using specialized hardware can analyze medical images such as X-rays, MRIs, and CT scans directly within hospital networks, identifying potential issues and prioritizing urgent cases for radiologist review. These systems maintain patient data privacy while providing faster results than cloud-based alternatives.
Wearable health monitors with embedded AI processors continuously track vital signs and can detect anomalies that might indicate health problems. By processing data locally, these devices can function without constant connectivity while preserving battery life and protecting sensitive health information.
Surgical robots with advanced AI capabilities assist surgeons with precision tasks, providing real-time guidance based on pre-operative imaging and intraoperative data. The low-latency processing enabled by specialized AI hardware is critical for these applications, where even milliseconds of delay could impact outcomes.
Industrial Automation and Manufacturing
Manufacturing facilities are increasingly deploying edge AI hardware to enhance quality control, optimize production processes, and implement predictive maintenance.
Vision systems powered by edge AI accelerators inspect products at high speed, identifying defects with greater accuracy than traditional computer vision approaches. By processing images directly on the factory floor, these systems can make real-time decisions to reject defective items without introducing delays in the production line.
Digital twins—virtual replicas of physical systems—are being enhanced with AI hardware to provide more accurate simulations and predictions. By combining sensor data with AI models running on specialized hardware, manufacturers can optimize production parameters in real-time and predict maintenance needs before equipment failures occur.
Collaborative robots (cobots) with embedded AI processors work alongside human employees, adapting their behavior based on real-time environmental awareness. The on-device processing capabilities allow these robots to respond instantly to human movements, ensuring safety while maximizing productivity.
Creative Industries and Content Creation
AI hardware is revolutionizing creative workflows in 2025, enabling new forms of content creation and production efficiencies.
Video production studios use AI accelerators to enhance footage in real-time, applying effects, color grading, and even generating background elements without the lengthy rendering times previously required. This allows directors and editors to see final-quality output during shooting, reducing the need for reshoots and post-production work.
Music production has been transformed by AI hardware that can generate accompaniments, suggest chord progressions, and even create entire backing tracks based on a simple melody input. These tools run locally on specialized AI hardware, allowing musicians to experiment without relying on cloud services or experiencing creative-breaking latency.
Game developers leverage edge AI to create more realistic non-player characters (NPCs) that adapt to player behavior in real-time. By running sophisticated AI models directly on gaming hardware, developers can create more immersive experiences without the limitations imposed by server-based AI.
How to Choose the Right AI Hardware
Factors to Consider When Purchasing
Selecting the appropriate AI hardware requires careful consideration of several key factors:
- Workload requirements: Different AI tasks demand different hardware capabilities. Image recognition, natural language processing, and reinforcement learning each have unique computational profiles.
- Performance needs: Consider whether your application requires real-time processing or can tolerate some latency. This will help determine whether edge processing, cloud computing, or a hybrid approach is most appropriate.
- Power constraints: For mobile or battery-powered applications, energy efficiency is critical. Look for hardware with high TOPS/watt ratings.
- Form factor: Physical size constraints may limit your hardware options, particularly for embedded applications.
- Development ecosystem: Consider the availability of software tools, frameworks, and pre-trained models compatible with your chosen hardware.
- Scalability: Evaluate whether the hardware can grow with your needs or if you'll need to replace it as your requirements evolve.
- Budget: Balance performance requirements against cost constraints, considering both initial purchase price and ongoing operational expenses.
Performance Metrics Explained
Understanding AI hardware performance metrics is essential for making informed decisions:
- TOPS (Tera Operations Per Second): Measures the number of operations a chip can perform per second. Higher TOPS generally indicates better performance for AI workloads, but this metric alone doesn't tell the complete story.
- TOPS/Watt: Indicates energy efficiency by measuring performance relative to power consumption. This is particularly important for edge devices and data centers concerned with energy costs.
- Precision support: AI hardware may support different numerical precisions (FP32, FP16, INT8, etc.). Lower precision generally enables faster computation but may reduce accuracy for some models.
- Memory bandwidth: The rate at which data can be read from or stored into memory, often a bottleneck for AI workloads.
- Inference latency: The time required to run a single inference pass through a model, critical for real-time applications.
- Model compatibility: Some hardware is optimized for specific model architectures or frameworks, which may limit flexibility.
When evaluating hardware based on these metrics, it's important to consider your specific use case rather than focusing solely on raw numbers.
Cost-Benefit Analysis
The cost of AI hardware extends beyond the initial purchase price. A comprehensive cost-benefit analysis should consider:
- Development time: Hardware with better development tools and ecosystem support may reduce time-to-market, offsetting higher initial costs.
- Energy costs: More efficient hardware can significantly reduce operational expenses, particularly for always-on applications or data center deployments.
- Maintenance and reliability: Consider the expected lifespan and reliability of the hardware, as well as the availability of support and replacement parts.
- Scalability costs: Evaluate whether scaling your solution will require linear hardware additions or if there are economies of scale.
- Training vs. inference: Some hardware excels at training but is less efficient for inference, or vice versa. Match your hardware to your primary workload.
- Cloud vs. edge costs: For some applications, the ongoing costs of cloud AI services may exceed the upfront cost of edge hardware over time.
By carefully weighing these factors against your specific requirements and constraints, you can identify the AI hardware solution that offers the best value for your use case.
The Future of AI Hardware: What's Next
Emerging Technologies on the Horizon
Several promising technologies are poised to reshape the AI hardware landscape in the coming years:
- Photonic computing: Using light instead of electricity to perform computations, photonic chips promise orders of magnitude improvements in speed and energy efficiency for certain AI workloads. Companies like Lightmatter and Luminous Computing are making significant progress in this area.
- In-memory computing: By performing computations directly within memory rather than shuttling data between memory and processors, in-memory computing architectures can dramatically reduce energy consumption and increase performance for AI tasks.
- 3D chip stacking: Advanced packaging technologies that stack multiple chips vertically are enabling higher performance and memory bandwidth while reducing the physical footprint of AI accelerators.
- Analog AI chips: By performing computations in the analog domain rather than digitally, these chips can achieve significant efficiency gains for certain neural network operations, though they face challenges related to precision and manufacturing.
- Biological and molecular computing: Though still largely experimental, computing systems inspired by or directly utilizing biological processes show promise for specific types of AI workloads.
Predictions for 2026 and Beyond
Based on current trends and emerging technologies, we can make several predictions about the future of AI hardware:
- Increased specialization: AI chips will become increasingly tailored to specific domains and applications, with custom silicon for everything from large language models to computer vision to scientific computing.
- Democratization of AI hardware: As manufacturing costs decrease and design tools improve, we'll see more companies developing their own custom AI accelerators rather than relying on general-purpose solutions from major vendors.
- Hybrid architectures: Future AI systems will increasingly combine different types of processors (CPUs, GPUs, NPUs, etc.) optimized for different aspects of AI workloads, with intelligent scheduling systems directing tasks to the most appropriate hardware.
- Edge-cloud continuum: The distinction between edge and cloud computing will blur, with seamless offloading of AI tasks between local devices and remote servers based on real-time conditions and requirements.
- AI-designed AI hardware: AI itself will play an increasingly important role in designing the next generation of AI chips, potentially discovering novel architectures that human engineers might not consider.
Challenges and Opportunities
The evolution of AI hardware faces several significant challenges:
- Energy consumption: As AI models continue to grow in size and complexity, their energy requirements threaten to become unsustainable without dramatic improvements in hardware efficiency.
- Supply chain constraints: The concentration of semiconductor manufacturing capacity and the complexity of chip design create potential bottlenecks in the AI hardware supply chain.
- Talent shortage: The specialized knowledge required to design and optimize AI hardware is in short supply, potentially limiting innovation.
- Standardization: The proliferation of custom AI accelerators creates challenges for software compatibility and developer productivity.
However, these challenges also present opportunities for innovation:
- Sustainable AI: Companies that can deliver high-performance AI with significantly lower energy requirements will have a competitive advantage as environmental concerns grow.
- Regional manufacturing: Efforts to diversify semiconductor manufacturing geographically could create new centers of AI hardware innovation.
- Automated design tools: Advances in chip design automation could help address the talent shortage while potentially leading to novel architectures.
- Software abstraction layers: Tools that allow developers to target multiple hardware platforms without code changes will become increasingly valuable in a fragmented hardware landscape.
Summary and Key Takeaways
The AI hardware landscape in 2025 is characterized by rapid innovation, increasing specialization, and a shift toward edge computing. Key takeaways from our exploration include:
- Hardware is strategic again: After years as a commodity, hardware has reemerged as a critical differentiator for AI capabilities.
- Edge AI is ascendant: The ability to process AI workloads locally, without cloud connectivity, is enabling new applications and addressing privacy concerns.
- Energy efficiency matters: As AI deployments scale, their environmental impact is driving innovation in more efficient hardware designs.
- Consumer AI hardware is maturing: From smart glasses to AI-enhanced headphones, consumer products are incorporating sophisticated AI capabilities in user-friendly forms.
- Developer platforms are diversifying: A growing ecosystem of development hardware is making AI more accessible to innovators across industries.
- Real-world applications are expanding: AI hardware is transforming everything from healthcare to manufacturing to creative industries.
- The future is specialized: The trend toward custom, application-specific AI accelerators will likely continue and accelerate.
For businesses, developers, and consumers navigating this complex landscape, staying informed about the latest hardware trends and capabilities is essential. By understanding the strengths, limitations, and appropriate applications of different AI hardware options, you can make strategic decisions that leverage these powerful technologies effectively.
As AI continues to transform industries and create new possibilities, the hardware that enables these advances will remain a critical foundation for innovation. Those who can harness the potential of specialized AI hardware will be well-positioned to lead in this new era of intelligent computing.
Frequently Asked Questions
What is the difference between NPUs, TPUs, and GPUs for AI processing?
NPUs (Neural Processing Units) are processors specifically designed for accelerating neural network workloads. They typically excel at inference tasks and are commonly found in smartphones, edge devices, and some server applications. NPUs are optimized for the specific mathematical operations used in neural networks, making them more energy-efficient for these tasks than general-purpose processors.
TPUs (Tensor Processing Units) are Google's custom-developed ASICs (Application-Specific Integrated Circuits) designed to accelerate machine learning workloads. They're optimized for Google's TensorFlow framework and excel at both training and inference for certain types of models. TPUs are available through Google Cloud and in edge form factors through the Coral platform.
GPUs (Graphics Processing Units) were originally designed for rendering graphics but have proven highly effective for AI workloads due to their parallel processing capabilities. NVIDIA's GPUs, enhanced with specialized tensor cores, remain the dominant hardware for training large AI models, though they typically consume more power than purpose-built AI accelerators.
Each has different strengths: GPUs offer flexibility and ecosystem support, TPUs excel at specific TensorFlow workloads, and NPUs typically offer the best energy efficiency for edge deployment.
How do I determine if I need edge AI hardware or cloud-based processing?
Consider edge AI hardware when:
- Low latency is critical (real-time applications)
- Privacy concerns require local data processing
- Your application needs to function without internet connectivity
- Bandwidth constraints make sending data to the cloud impractical
- Operational costs of continuous cloud processing are prohibitive
Cloud-based AI processing may be more appropriate when:
- Your models are too large or complex for edge hardware
- You need to process massive datasets
- Your workloads are batch-oriented rather than real-time
- You require flexibility to scale processing up or down quickly
- Your application already has cloud dependencies
Many sophisticated AI systems in 2025 use a hybrid approach, performing initial processing at the edge and selectively sending data to the cloud for more complex analysis when necessary.
What are the key performance metrics I should look for when comparing AI hardware?
Beyond the metrics discussed in the "Performance Metrics Explained" section, consider:
- Model compatibility: Ensure the hardware supports the specific models and frameworks you intend to use.
- Batch processing efficiency: Some hardware performs better with batched inputs, which may or may not align with your use case.
- Quantization support: The ability to run models at lower precision can significantly improve performance but may affect accuracy.
- Memory capacity: Insufficient memory can become a bottleneck regardless of computational power.
- Software optimization tools: The availability of tools to optimize your models for specific hardware can dramatically impact real-world performance.
Remember that benchmark numbers alone don't tell the complete story—test your specific workloads on candidate hardware whenever possible before making significant investments.
How is AI hardware addressing environmental sustainability concerns?
AI hardware manufacturers are addressing sustainability through several approaches:
- Improved energy efficiency: New chip designs focus on maximizing TOPS/watt, reducing the energy required for AI computations.
- Specialized accelerators: Purpose-built hardware can be orders of magnitude more efficient than general-purpose processors for specific AI tasks.
- Advanced cooling technologies: Innovations in cooling, particularly liquid cooling, are reducing the energy overhead of thermal management.
- Optimized data center design: AI-specific data centers are being designed from the ground up for efficiency, with power usage effectiveness (PUE) as a key metric.
- Carbon-aware computing: Some AI systems now incorporate awareness of the carbon intensity of available electricity, scheduling intensive workloads during periods of lower carbon emissions.
- Hardware recycling programs: Major manufacturers are implementing programs to recycle or refurbish AI hardware, reducing electronic waste.
- Renewable energy investments: Companies with large AI infrastructure are increasingly investing directly in renewable energy projects to offset their carbon footprint.
What should I consider when future-proofing my AI hardware investments?
To maximize the longevity and value of your AI hardware investments:
- Prioritize flexibility: Choose platforms with broad model and framework support rather than those optimized for a single approach that may become obsolete.
- Consider modularity: Systems that allow component upgrades can extend useful life without complete replacement.
- Evaluate software support commitments: Look for vendors with a track record of long-term software support and regular updates.
- Assess scalability: Ensure your chosen platform can scale with your needs, either through clustering or seamless migration paths to more powerful hardware.
- Look beyond raw performance: Features like security, reliability, and developer experience often determine long-term value more than peak performance metrics.
- Consider total cost of ownership: Factor in energy costs, maintenance requirements, and potential integration challenges when comparing options.
- Hedge your bets: For critical applications, maintaining compatibility with multiple hardware platforms can provide insurance against vendor-specific risks.